Tune in for a free, live virtual hands-on lab...
What is the Difference Between a Data Lake and a Data Warehouse?
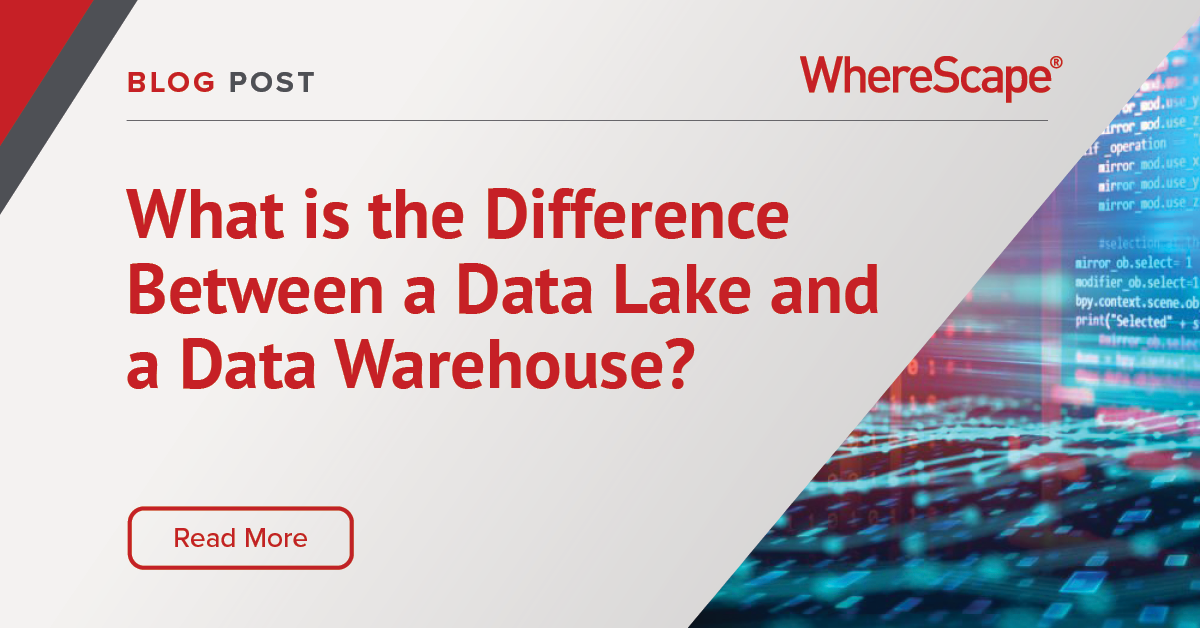
The Data warehouse and data lake are the two leading solutions for enterprise data management. While data warehouses and data lakes might share some overlapping features and use cases, there are fundamental differences in the data management philosophies, design characteristics, and ideal use conditions for each of these platforms.
In this blog post, we take a closer look at the key differences between the data lake and data warehouse platform, and how to choose the right one for your business.
What is a Data Warehouse?
A data warehouse is designed for highly structured data generated by business applications. It brings all your data together and stores it in a structured manner. It is a data management platform that provides business intelligence for structured operational data, usually from a relational database management system (RDBMS). It ingests structured data with predefined schema, then connects that data to downstream analytical tools that support business intelligence (BI) initiatives.
Data warehouses support sequential ETL operations, where data flows in a waterfall model from the raw data format to a fully transformed set, optimized for fast performance. This platform relies on the structure of data to support high-performance SQL (Structured Query Language) operations. Some newer data warehouses support semi-structured data such as JSON, Parquet, and XML files.
It is possible to automate the design, development and production of a data warehouse. Organizations have seen projects estimated to take years reduced to months and sometimes weeks. WhereScape provides data warehouse automation software to achieve these goals.
What is a Data Lake?
A data lake is a centralized data repository where structured, semi-structured, and unstructured data from a variety of sources can be stored in their raw format. It helps eliminate data silos by acting as a single landing zone from multiple sources.
A data lake is ideal for machine learning use cases. It provides SQL-based access to data and native support for programmatic distributed data processing frameworks like Apache Spark and Tensorflow through languages such as Python, Scala, Java, and more. It supports native streaming, where streams of data are processed and made available for analytics as they arrive.
The key purpose of a data lake is to make organizational data from various sources accessible to different end-users like business analysts, data engineers, data scientists, product managers, executives, etc, to leverage insights in a cost-effective manner for improved business performance.
Choosing the right platform for your organization
Both data warehouse and data lake solutions are not mutually exclusive. Neither a data lake nor a data warehouse on its own comprises a data and analytics strategy, but both solutions can be used together.
The data warehouse model is all about functionality and performance. It ingests data from RDBS, transforms it into something useful, then pushes the transformed data to downstream BI and analytics applications. These functions are essential, but the data warehouse paradigm of schema-on-write, tightly coupled storage/compute, and reliance on predefined use cases makes the data warehouse the wrong choice for big, multi-structured data or multi-model capabilities.
In contrast, a data lake is more suited to meeting the demands of a big data world: schema-on-read, loosely coupled storage/compute, and flexible use cases that combine to drive innovation by reducing the time, cost, and complexity of data management. However, without data warehouse functionality, a data lake can become a data swamp.
WhereScape can automate the development and maintenance of your data warehouse. Through two products, WhereScape RED and WhereScape 3D, your organization can achieve its data warehouse goals in a fraction of the time as opposed to developing manually.
If you would like to see WhereScape in action, please request a demo.
ETL vs ELT: What are the Differences?
In working with hundreds of data teams through WhereScape’s automation platform, we’ve seen this debate evolve as businesses modernize their infrastructure. Each method, ETL vs ELT, offers a unique pathway for transferring raw data into a warehouse, where it can be...
Dimensional Modeling for Machine Learning
Kimball’s dimensional modeling continues to play a critical role in machine learning and data science outcomes, as outlined in the Kimball Group’s 10 Essential Rules of Dimensional Modeling, a framework still widely applied in modern data workflows. In a recent...
Automating Data Vault in Databricks | WhereScape Recap
Automating Data Vault in Databricks can reduce time-to-value by up to 70%—and that’s why we hosted a recent WhereScape webinar to show exactly how. At WhereScape, modern data teams shouldn't have to choose between agility and governance. That's why we hosted a live...
WhereScape Recap: Highlights From Big Data & AI World London 2025
Big Data & AI World London 2025 brought together thousands of data and AI professionals at ExCeL London—and WhereScape was right in the middle of the action. With automation taking center stage across the industry, it was no surprise that our booth and sessions...
Why WhereScape is the Leading Solution for Healthcare Data Automation
Optimizing Healthcare Data Management with Automation Healthcare organizations manage vast amounts of medical data across EHR systems, billing platforms, clinical research, and operational analytics. However, healthcare data integration remains a challenge due to...
WhereScape Q&A: Your Top Questions Answered on Data Vault and Databricks
During our latest WhereScape webinar, attendees had fantastic questions about Data Vault 2.0, Databricks, and metadata automation. We’ve compiled the best questions and answers to help you understand how WhereScape streamlines data modeling, automation, and...
What is Data Fabric? A Smarter Way for Data Management
As of 2023, the global data fabric market was valued at $2.29 billion and is projected to grow to $12.91 billion by 2032, reflecting the critical role and rapid adoption of data fabric solutions in modern data management. The integration of data fabric solutions...
Want Better AI Data Management? Data Automation is the Answer
Understanding the AI Landscape Imagine losing 6% of your annual revenue—simply due to poor data quality. A recent survey found that underperforming AI models, built using low-quality or inaccurate data, cost companies an average of $406 million annually. Artificial...
RED 10: The ‘Git Friendly’ Revolution for CI/CD in Data Warehousing
For years, WhereScape RED has been the engine that powers rapidly built and high performance data warehouses. And while RED 10 has quietly empowered organizations since its launch in 2023, our latest 10.4 release is a game changer. We have dubbed this landmark update...
The Assembly Line for Your Data: How Automation Transforms Data Projects
Imagine an old-fashioned assembly line. Workers pass components down the line, each adding their own piece. It’s repetitive, prone to errors, and can grind to a halt if one person falls behind. Now, picture the modern version—robots assembling products with speed,...
Related Content
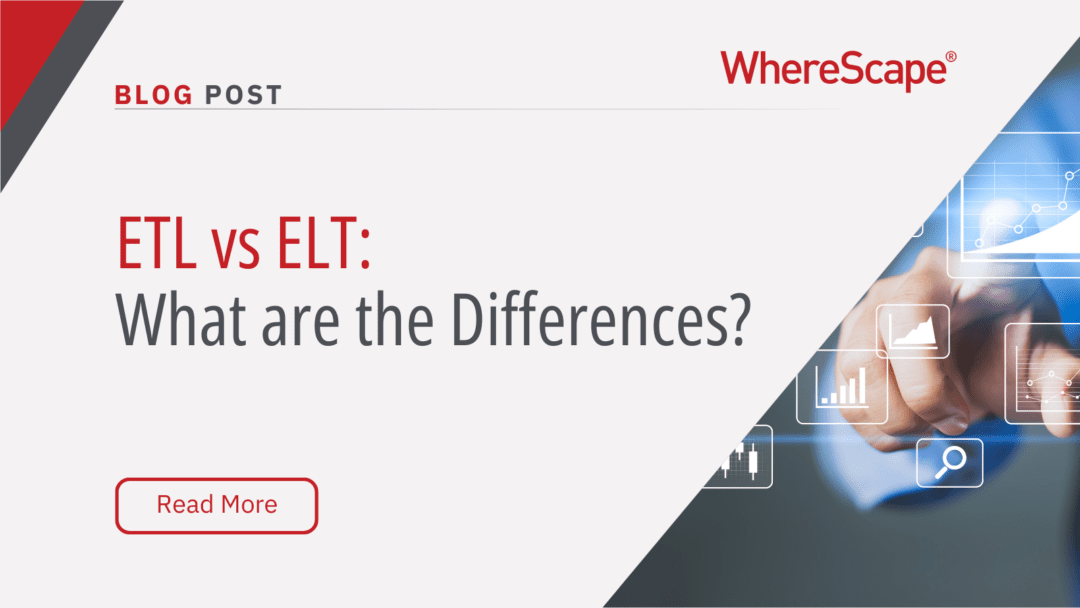
ETL vs ELT: What are the Differences?
In working with hundreds of data teams through WhereScape’s automation platform, we’ve seen this debate evolve as businesses modernize their infrastructure. Each method, ETL vs ELT, offers a unique pathway for transferring raw data into a warehouse, where it can be...
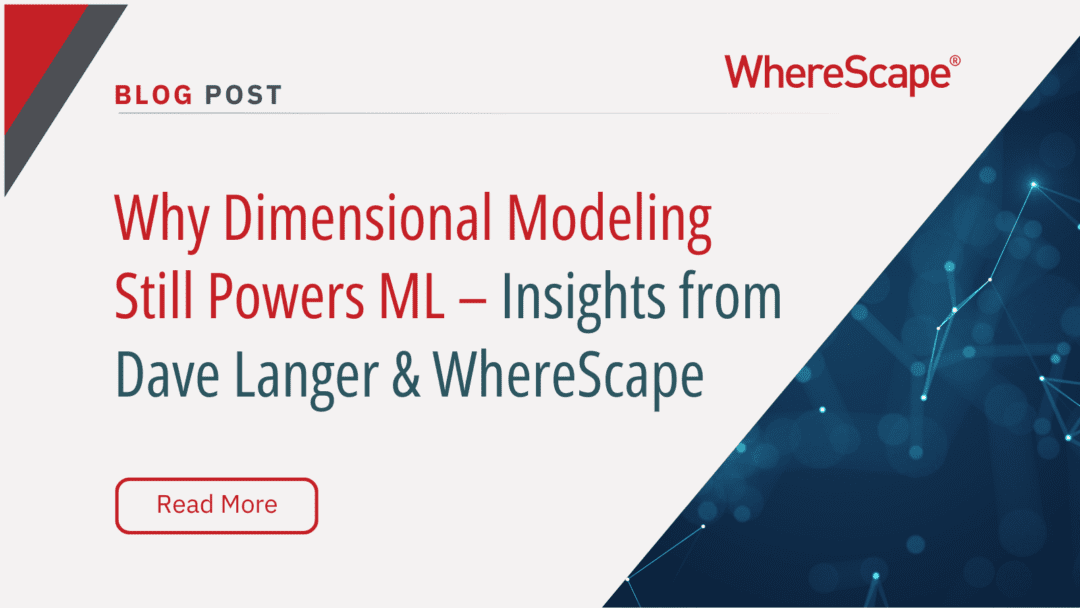
Dimensional Modeling for Machine Learning
Kimball’s dimensional modeling continues to play a critical role in machine learning and data science outcomes, as outlined in the Kimball Group’s 10 Essential Rules of Dimensional Modeling, a framework still widely applied in modern data workflows. In a recent...
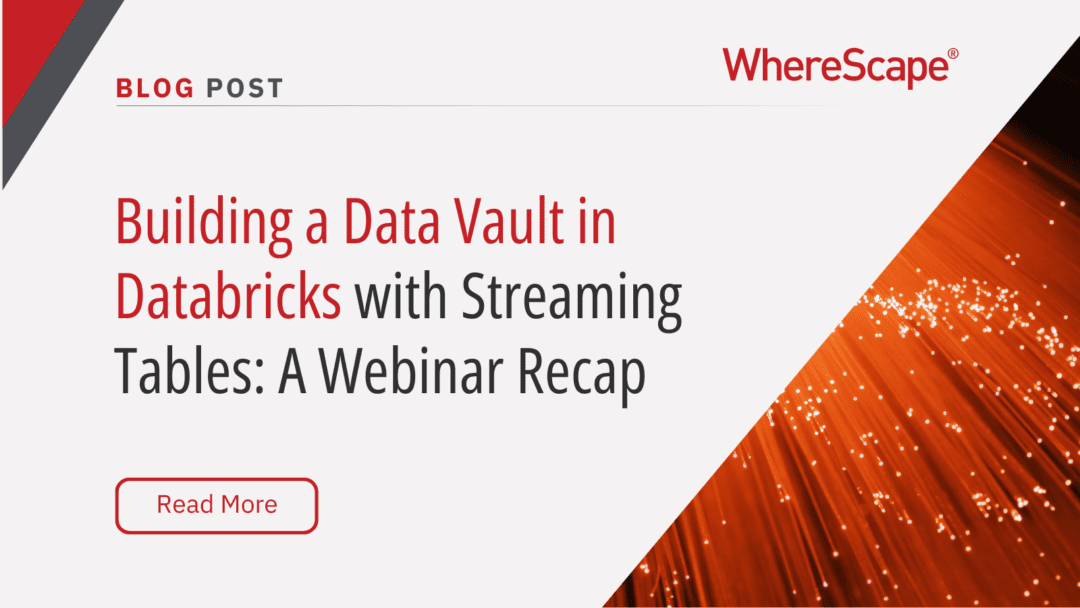
Automating Data Vault in Databricks | WhereScape Recap
Automating Data Vault in Databricks can reduce time-to-value by up to 70%—and that’s why we hosted a recent WhereScape webinar to show exactly how. At WhereScape, modern data teams shouldn't have to choose between agility and governance. That's why we hosted a live...
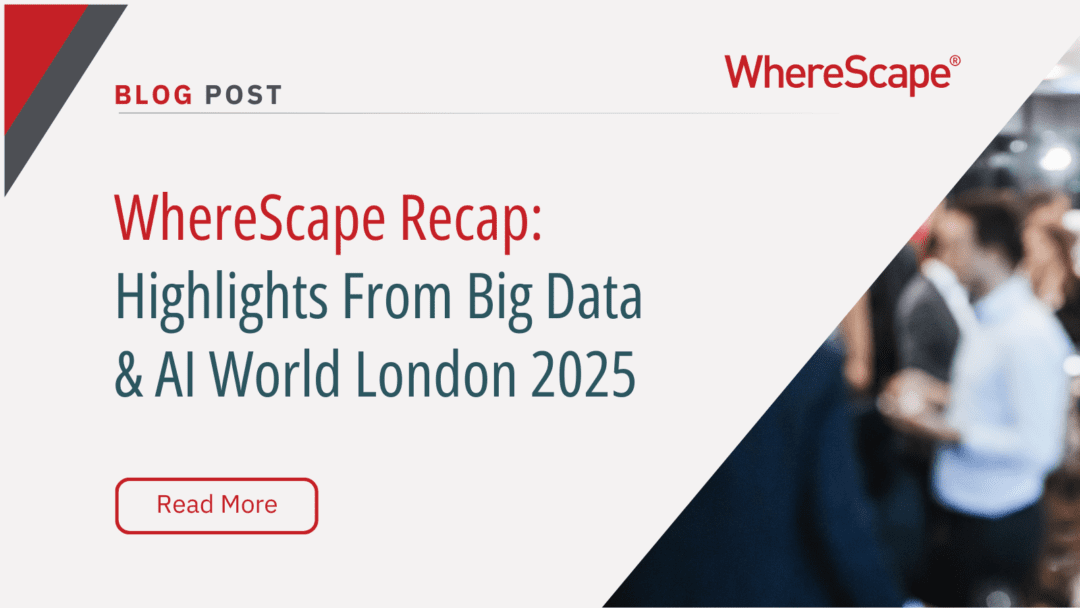
WhereScape Recap: Highlights From Big Data & AI World London 2025
Big Data & AI World London 2025 brought together thousands of data and AI professionals at ExCeL London—and WhereScape was right in the middle of the action. With automation taking center stage across the industry, it was no surprise that our booth and sessions...