Accelerate your path to a fully functioning data...
What is Data Analytics?
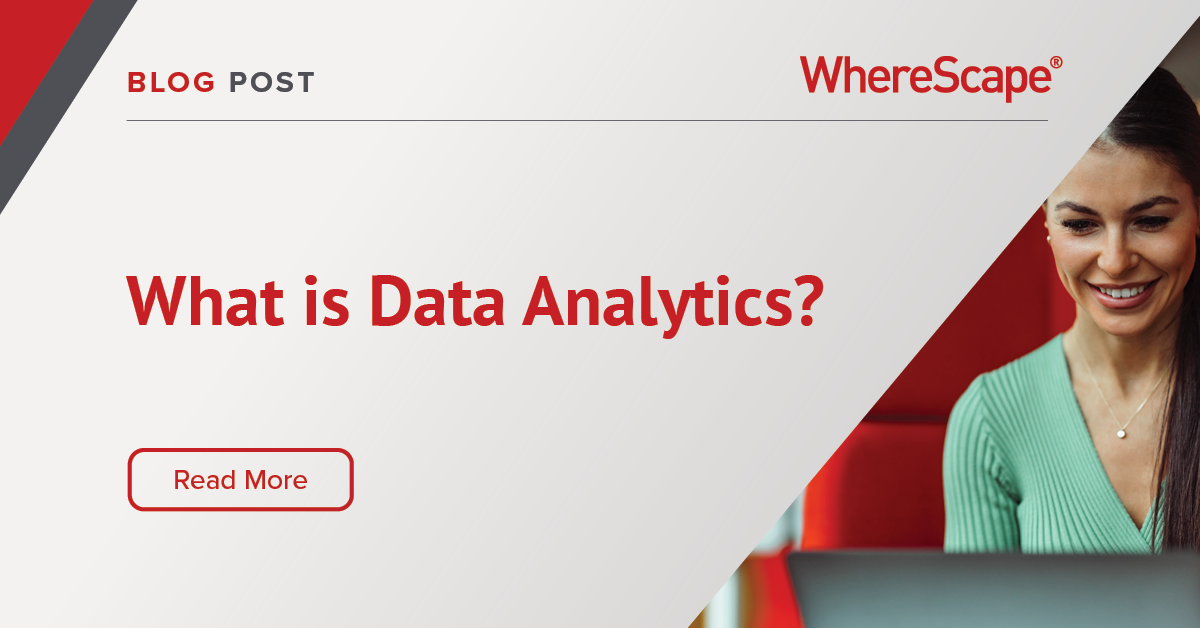
Data analytics is a process of analyzing raw data in order to draw out meaningful, actionable insights. These insights are then used to inform and drive smart business decisions. Data analytics technologies and techniques are widely used in commercial industries to enable organizations to make more-informed business decisions. For example, manufacturing companies often record the runtime, downtime, and work queue for various machines and then analyze the data to better plan the workloads so the machines operate closer to peak capacity. Scientists and researchers also use analytics tools to verify and disapprove scientific models, theories, and hypotheses.
The ultimate goal of data analytics is to boost business performance. These initiatives can help businesses increase revenue, improve operational efficiency, optimize marketing campaigns and bolster customer service efforts. It also enables organizations to respond quickly to emerging market trends and gain a competitive advantage over business rivals.
Types of data analytics
There are four main types of data analysis:
- Descriptive analytics: This describes what has happened over a given period of time.
- Diagnostic analytics: Focuses more on why something happened. This involves more diverse data inputs and a bit of hypothesizing.
- Predictive analytics: This estimates the likelihood of a future outcome based on historical data and probability theory, and while it can never be completely accurate, it does eliminate much of the guesswork from key business decisions. It can be used to forecast all sorts of outcomes – from what products will be most popular at a certain time, to how much the company revenue is likely to increase or decrease in a given period.
- Prescriptive analytics: This helps answer questions about what should be done. When conducting prescriptive analysis, data analysts will consider a range of possible scenarios and assess the different actions the company might take. Prescriptive analytics techniques rely on machine learning strategies that can find patterns in large datasets. By analyzing past decisions and events, the likelihood of different outcomes can be estimated.
Why is Data Analytics Important?
Data analytics helps businesses optimize their performance. By implementing it into the business model, companies can help reduce costs by identifying more efficient ways of doing business. A company can also use data analytics to make better business decisions and help analyze customer trends and satisfaction, which can lead to better products and services.
Furthermore, data analytics is used to make faster and more informed decisions, reduce overall business costs, and optimize processes and operations. In more specific terms data analytics might be used:
- To predict futures and purchasing behaviors
- For security purposes, for instance, to predict, to detect, and prevent fraud within insurance and banking industries
- To optimize marketing efforts through more accurate targeting and personalization
- To boost customer acquisition and retention
- To boost customer engagement on social media
- To develop risk management solutions
- To increase supply chain efficiency
Conclusion
Organizations that approach data analytics with a focused vision can drive digital transformation, improve customer experience, and create a data-driven company culture. Leveraging data analytics, organizations can identify new business opportunities and use insights to prioritize actions and create a new source of revenue. Data analytics programs within the organizations are evolving rapidly as digital transformation and data-driven organizations are becoming more of a priority.
What is a Cloud Data Warehouse?
As organizations increasingly turn to data-driven decision-making, the demand for cloud data warehouses continues to rise. The cloud data warehouse market is projected to grow significantly, reaching $10.42 billion by 2026 with a compound annual growth rate (CAGR) of...
Simplify Cloud Migrations: Webinar Highlights from Mike Ferguson
Migrating your data warehouse to the cloud might feel like navigating uncharted territory, but it doesn’t have to be. In a recent webinar that we recently hosted, Mike Ferguson, CEO of Intelligent Business Strategies, shared actionable insights drawn from his 40+...
2025 Data Automation Trends: Shaping the Future of Speed, Scalability, and Strategy
As we step into 2025, data automation isn’t just advancing—it’s upending conventions and resetting standards. Leading companies now treat data as a powerful collaborator, fueling key business decisions and strategic foresight. At WhereScape, we’re tuned into the next...
Building Smarter with a Metadata-Driven Approach
Think of building a data management system as constructing a smart city. In this analogy, the data is like the various buildings, roads, and infrastructure that make up the city. Each structure has a specific purpose and function, just as each data point has a...
Your Guide to Online Analytical Processing (OLAP) for Business Intelligence
Streamline your data analysis process with OLAP for better business intelligence. Explore the advantages of Online Analytical Processing (OLAP) now! Do you find it hard to analyze large amounts of data quickly? Online Analytical Processing (OLAP) is designed to answer...
Mastering Data Warehouse Design, Optimization, And Lifecycle
Building a data warehouse can be tough for many businesses. A data warehouse centralizes data from many sources. This article will teach you how to master data warehouse design, optimization, and lifecycle. Start improving your data strategy today. Key Takeaways Use...
Revisiting Gartner’s First Look at Data Warehouse Automation
At WhereScape, we are delighted to revisit Gartner’s influential technical paper, Assessing the Capabilities of Data Warehouse Automation (DWA), published on February 8, 2021, by analyst Ramke Ramakrishnan. This paper marked a significant milestone for the data...
Unveiling WhereScape 3D 9.0.5: Enhanced Flexibility and Compatibility
The latest release of WhereScape 3D is here, and version 9.0.5 brings a host of updates designed to make your data management work faster and smoother. Let’s dive into the new features... Online Documentation for Enhanced Accessibility With the user guide now hosted...
What Makes A Really Great Data Model: Essential Criteria And Best Practices
By 2025, over 75% of data models will integrate AI—transforming the way businesses operate. But here's the catch: only those with robust, well-designed data models will reap the benefits. Is your data model ready for the AI revolution?Understanding what makes a great...
Guide to Data Quality: Ensuring Accuracy and Consistency in Your Organization
Why Data Quality Matters Data is only as useful as it is accurate and complete. No matter how many analysis models and data review routines you put into place, your organization can’t truly make data-driven decisions without accurate, relevant, complete, and...
Related Content
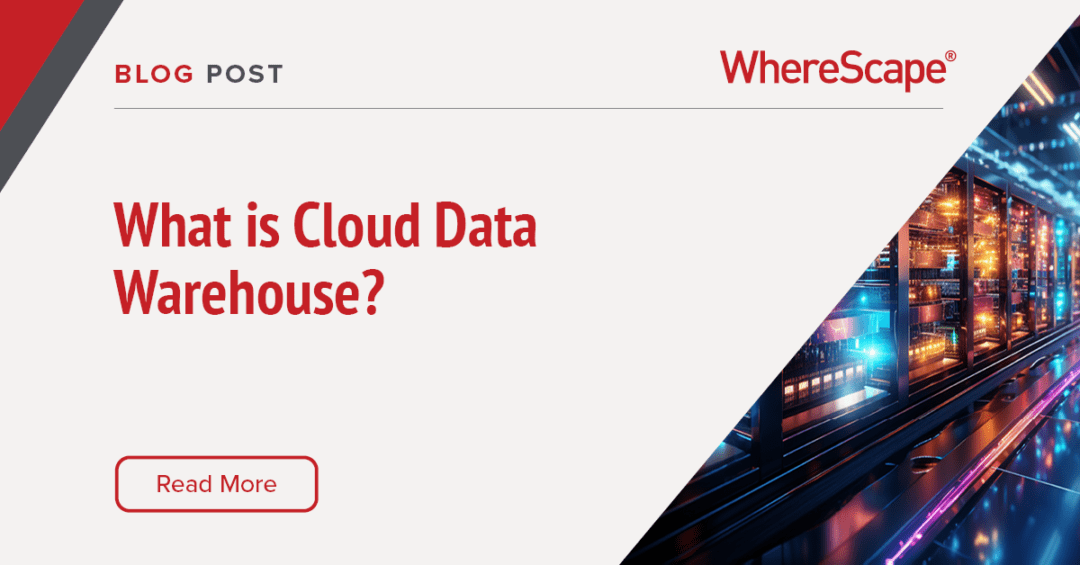
What is a Cloud Data Warehouse?
A cloud data warehouse is an advanced database service managed and hosted over the internet.
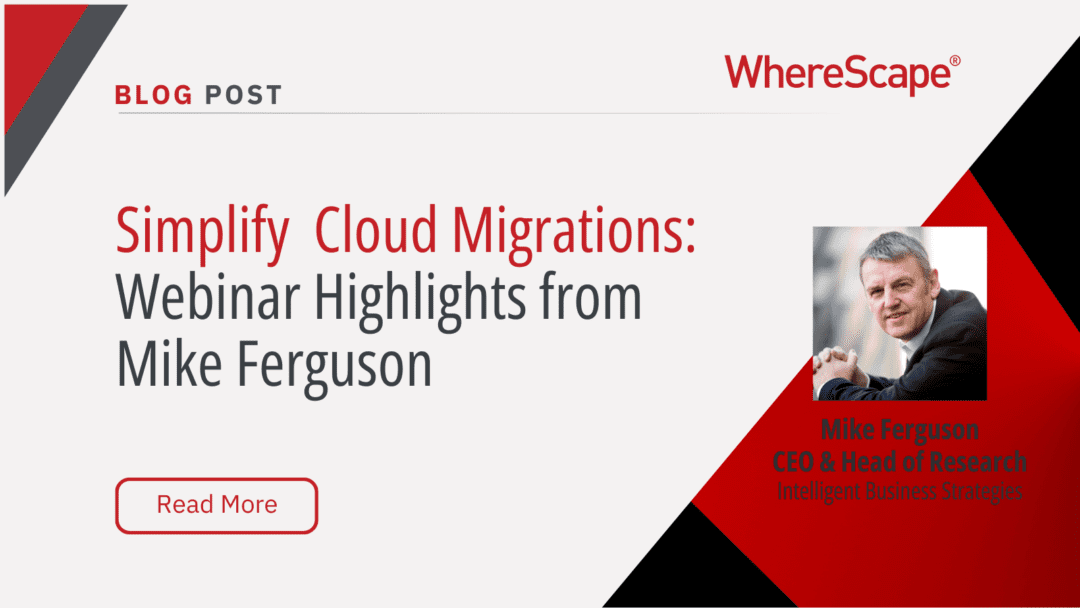
Simplify Cloud Migrations: Webinar Highlights from Mike Ferguson
Migrating your data warehouse to the cloud might feel like navigating uncharted territory, but it doesn’t have to be. In a recent webinar that we recently hosted, Mike Ferguson, CEO of Intelligent Business Strategies, shared actionable insights drawn from his 40+...
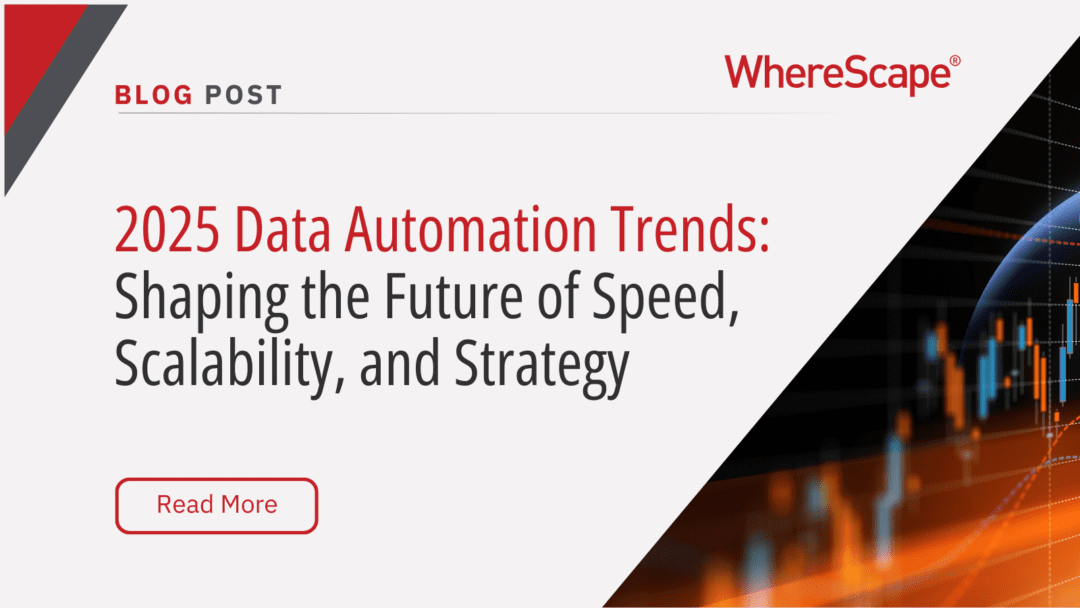
2025 Data Automation Trends: Shaping the Future of Speed, Scalability, and Strategy
As we step into 2025, data automation isn’t just advancing—it’s upending conventions and resetting standards. Leading companies now treat data as a powerful collaborator, fueling key business decisions and strategic foresight. At WhereScape, we’re tuned into the next...
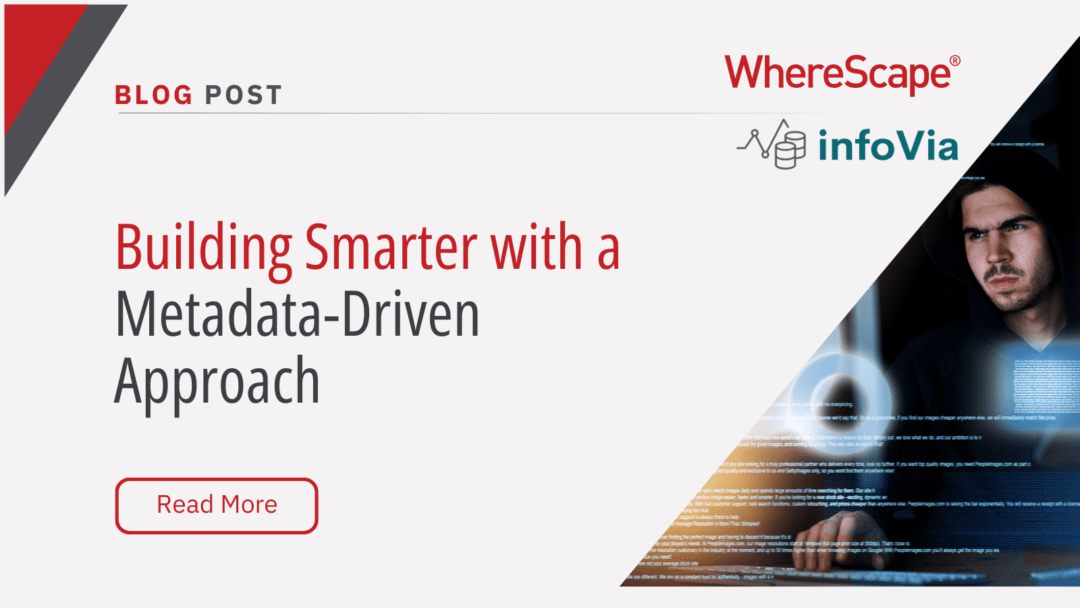
Building Smarter with a Metadata-Driven Approach
Think of building a data management system as constructing a smart city. In this analogy, the data is like the various buildings, roads, and infrastructure that make up the city. Each structure has a specific purpose and function, just as each data point has a...