Tune in for a free, live virtual hands-on lab...
Understanding Data Vault 2.0
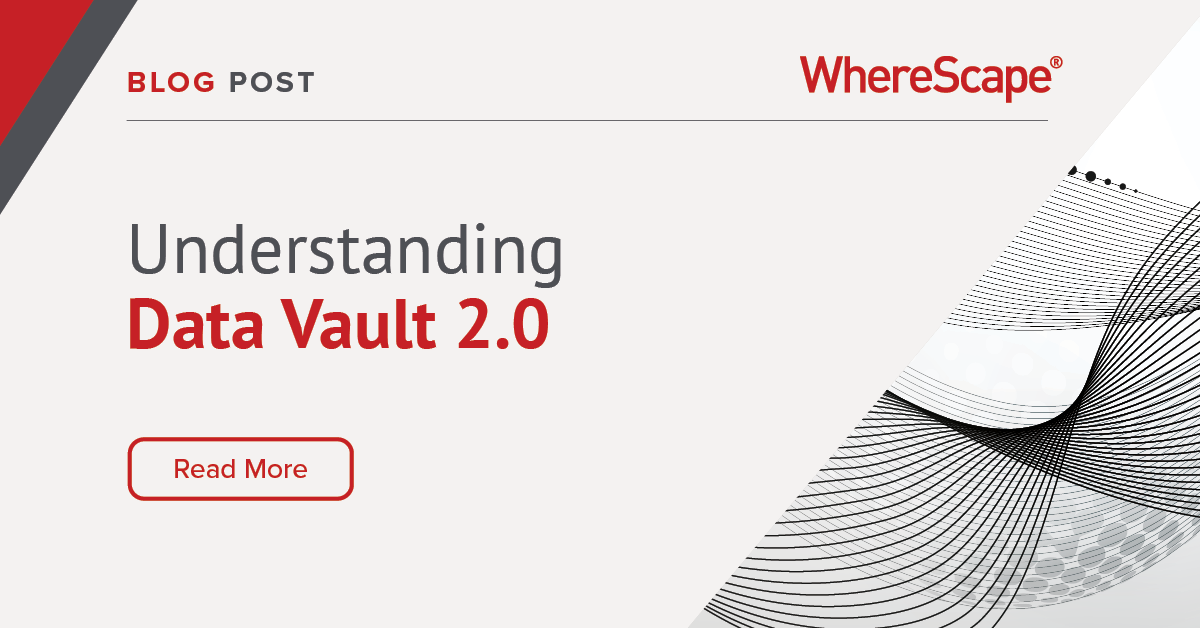
Data Vault Modeling
Implementing a data vault as your Data Modeling approach has many advantages, such as flexibility, scalability, and efficiency. But along with that, one must be aware of the challenges that come along with that. Data vault modeling leads to a significantly larger number of data objects like tables and columns because a data vault separates information types. To understand the benefits of auditability and adaptability with Data Vault 2.0, refer to our blog: Unlocking the Benefits of Auditability and Adaptability with Data Vault 2.0.
Data Vault Architecture
Data vault modeling leads to a significantly larger number of data objects like tables and columns because a data vault separates information types. This means that the modeling process can be larger and will involve more tasks to establish a flexible and detailed data model with all its components.
Data Vault 2.0 Methodology
The Data Vault 2.0 was designed as an “agile” data warehouse that can accommodate, change and support a constantly evolving view of enterprise data.
Data Vault 2.0 has become the standard in building large, scalable, and flexible data warehouses. It describes a wide-ranging architecture that encompasses metadata, audit, provenance, data loads, and master data management. At its core is a modeling technique that separates structural information from attributes by arranging data into one of three types of table: hubs (business entities), links (relationships), and satellites (attributes). This model provides a malleable layer that rests between staging and reporting layers in a data warehouse architecture.
Data Vault Solutions
Deferring design decisions
This approach makes it easier to perform common data warehouse design ‘additive’ tasks such as adding attributes, refactoring entity relationships, incorporating new sources and deprecating older ones, and building them on existing structures.
A vault model also provides separation between “raw” ingested data and the “business” data that has domain-specific rules applied to it. This is advantageous in cases where you need to frequently ingest new data sources, therefore giving you a lot more flexibility to make changes to entities and relationships without them cascading across the entire model.
Audit, performance, and automation
Apart from design flexibility, data vault modeling also offers solutions for auditing, data provenance, and loading speed. You can track where each data point is coming from as it breaks record sources into every separate entity, relationship, and attribute allowing you to retrieve the state of your data as it was understood at a particular point in time.
You can include audit information in the design of every item in order to trace information such as load time and load duration. To better understand how data warehouse automation can benefit your Data Vault 2.0 projects, see our blog: What Exactly Is Data Warehouse Automation, and Why Do I Want It?
Escalating complexity
Although Data Vault has these advantages, the downside is that you will have to learn to deal with escalating internal complexity.
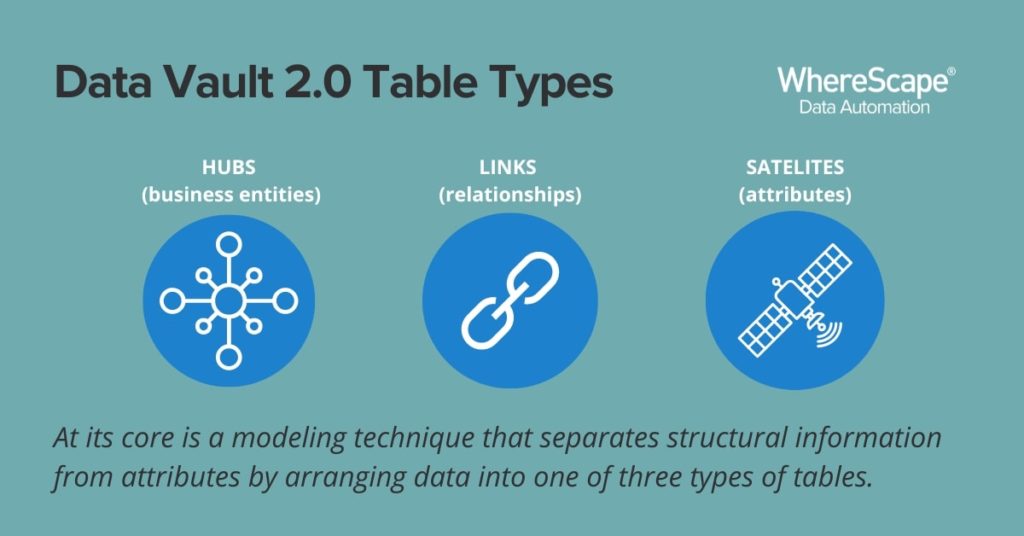
Raw Data Vault vs Business Data Vault
The basic concepts of hubs, satellites, and links are easy enough, but one needs to learn to differentiate between the “raw” vault of “hard” rules and the “business” vault of “soft” rules. The large number of tables involved in a vault database can be intimidating, compared to the other dimensions and relational models. In operational terms, this is where Data Vault 2.0 starts to suffer compared to relational or dimensional models.
Data Vault 2.0 should be treated carefully. You should try to implement it with proper training and consultancy support from people with some practical experience. Making the correct decisions early is critical to building a successful data vault.
The Nuances
While adopting the Data Vault 2.0, you will spend a lot of time explaining the technical and non-technical nuances to stakeholders. Compared with Kimball and Inmon, Data Vault 2.0 is regarded as an outlier since it lacks the widespread acceptance enjoyed by others.
The inherent abstraction in data vault modeling makes the data in the warehouse difficult to understand by those who have not been trained in the technique. Data Vault 2.0 may not be a good fit for enterprise-wide data governance initiatives that need to engage with a wide range of stakeholders.
Data Vault 2.0 Use Cases
Data Vault 2.0 is a better fit for scenarios where you have multiple, frequently changing source systems integrating into your enterprise warehouse. Data Vault 2.0 is about providing scalability and managing change. In cases where you have less than a dozen predictable sources, it may not be recommended for you.
Most importantly, you need to show patience and commitment as it can take time for the real benefits of the accumulated data in a vault to become more obvious.
Data Vault 2.0 Implementation
Data Vault 2.0 is increasingly becoming popular as a method for the design and building of Data Warehouses but, there are some potential pitfalls.
Let’s take a look at some common pitfalls in the Data Vault projects.
- Modeling without thinking: When crafting a data model, one needs to keep in mind the context. There are no rights or wrongs in data modeling. There may be different answers to different projects, depending on the analyst. For example, a modeler may make decisions early about entities such as customers. Many projects need to reverse some decisions as a part of their course correction. Thanks to Data Vault 2.0, its agility makes it easier for users to review the models.
- Applying standards: There are sets of standards for Data Vault 2.0 projects that have been developed over the years across many use cases in a plethora of industries. However, Data Warehouse professionals at times discount some of the standards before they have a complete understanding of why they are there. Unfortunately, the consequences of not following standards may not become clear immediately. With Data Vault 2.0 certification training, users can learn why the standards are applied and know when to flex them.
- Start simple and build out: Due to the management’s pressure, the first project selected is often too ambitious. Therefore, one must be very careful when selecting the first project. It might be better to select a part of a bigger reporting set because businesses can often find surprising value in a subset of data. Replicating a well-selected report can add considerable value to the data. From an experience point of view, selecting the first project requires careful expectation management.
Data Vault Express
WhereScape Data Vault Express removes the complexity inherent in data vault development, allowing you to automate the entire data vault lifecycle to deliver data vault solutions to the business faster, at lower cost and with less risk.
WhereScape Data Automation
WhereScape eliminates the risks in data projects and accelerates time to production to help organizations adapt better to changing business needs. Book a demo to see what you can achieve with WhereScape.
WhereScape at TDWI Munich: Automate Data Vault on Databricks
WhereScape at TDWI Munich 2025: Automate a Full Data Vault on Databricks in Just 45 Minutes June 24–26, 2025 | MOC Munich, Germany As data complexity grows and business demands accelerate, scalable and governed data architectures are no longer optional—they're...
What Is OLAP? Online Analytical Processing for Fast, Multidimensional Analysis
Streamline your data analysis process with OLAP for better business intelligence. Explore the advantages of Online Analytical Processing (OLAP) now! Do you find it challenging to analyze large volumes of data swiftly? A Forrester study reveals that data teams spend...
Build AI-Ready Data: Visit WhereScape at AI & Big Data Expo
June 4–5, 2025 | Booth 202 | Santa Clara Convention Center As organizations scale their artificial intelligence and analytics capabilities, the demand for timely, accurate, governed, and AI-ready data has become a strategic priority. According to Gartner, through...
Automating Star Schemas in Microsoft Fabric: A Webinar Recap
From Data Discovery to Deployment—All in One Workflow According to Gartner, data professionals dedicate more than half of their time, 56%, to operational tasks, leaving only 22% for strategic work that drives innovation. This imbalance is especially apparent when...
What is a Data Model? How Structured Data Drives AI Success
What is a data model? According to the 2020 State of Data Science report by Anaconda, data scientists spend about 45% of their time on data preparation tasks, including cleaning and loading data. Without well-structured data, even the most advanced AI systems can...
ETL vs ELT: What are the Differences?
In working with hundreds of data teams through WhereScape’s automation platform, we’ve seen this debate evolve as businesses modernize their infrastructure. Each method, ETL vs ELT, offers a unique pathway for transferring raw data into a warehouse, where it can be...
Dimensional Modeling for Machine Learning
Kimball’s dimensional modeling continues to play a critical role in machine learning and data science outcomes, as outlined in the Kimball Group’s 10 Essential Rules of Dimensional Modeling, a framework still widely applied in modern data workflows. In a recent...
Automating Data Vault in Databricks | WhereScape Recap
Automating Data Vault in Databricks can reduce time-to-value by up to 70%—and that’s why we hosted a recent WhereScape webinar to show exactly how. At WhereScape, modern data teams shouldn't have to choose between agility and governance. That's why we hosted a live...
WhereScape Recap: Highlights From Big Data & AI World London 2025
Big Data & AI World London 2025 brought together thousands of data and AI professionals at ExCeL London—and WhereScape was right in the middle of the action. With automation taking center stage across the industry, it was no surprise that our booth and sessions...
Why WhereScape is the Leading Solution for Healthcare Data Automation
Optimizing Healthcare Data Management with Automation Healthcare organizations manage vast amounts of medical data across EHR systems, billing platforms, clinical research, and operational analytics. However, healthcare data integration remains a challenge due to...
Related Content
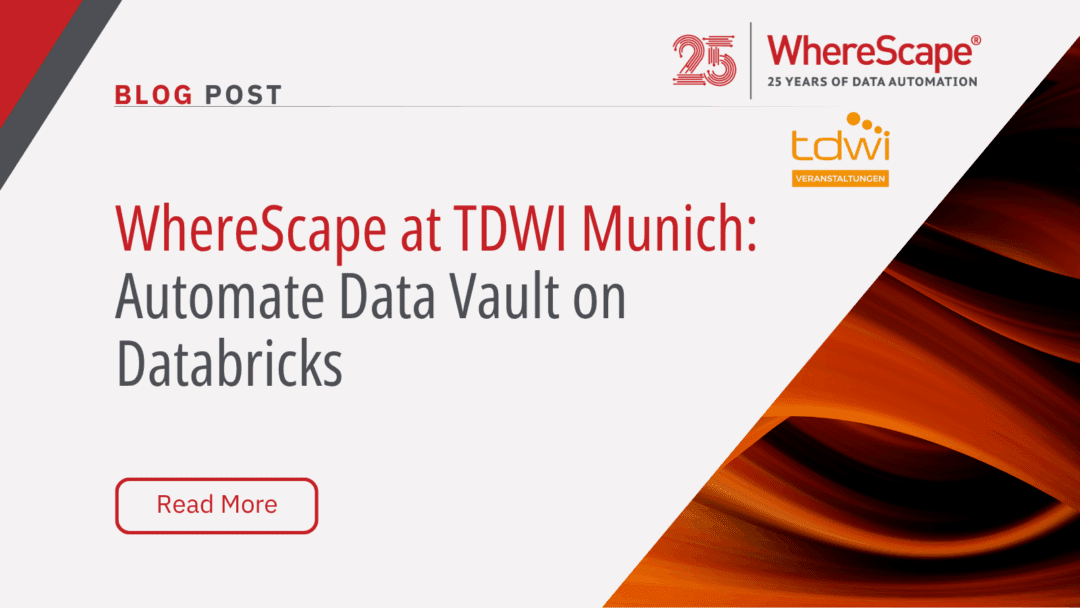
WhereScape at TDWI Munich: Automate Data Vault on Databricks
WhereScape at TDWI Munich 2025: Automate a Full Data Vault on Databricks in Just 45 Minutes June 24–26, 2025 | MOC Munich, Germany As data complexity grows and business demands accelerate, scalable and governed data architectures are no longer optional—they're...
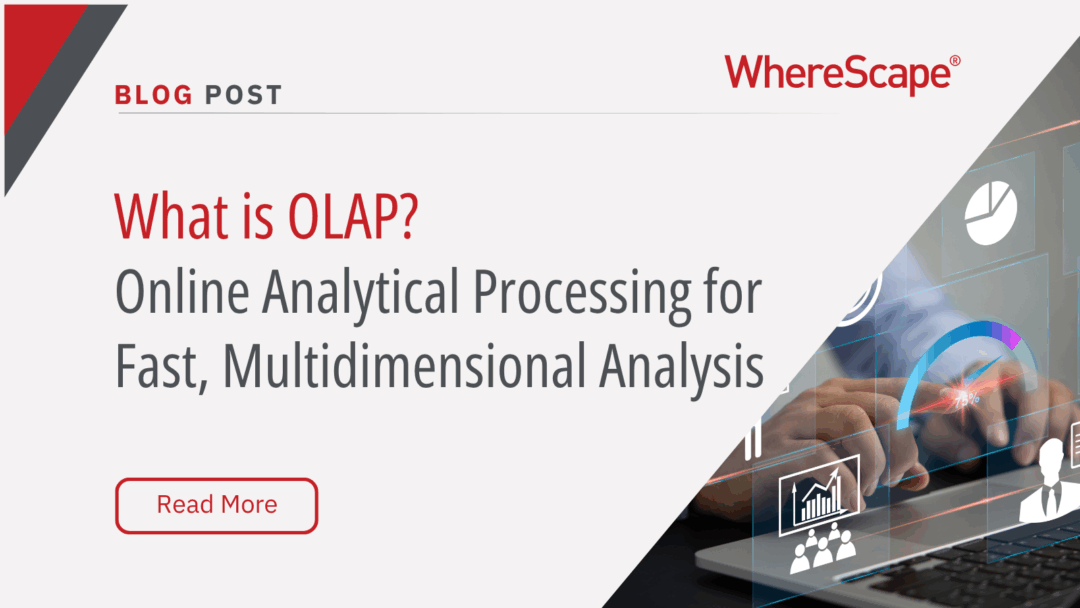
What Is OLAP? Online Analytical Processing for Fast, Multidimensional Analysis
Streamline your data analysis process with OLAP for better business intelligence. Explore the advantages of Online Analytical Processing (OLAP) now! Do you find it challenging to analyze large volumes of data swiftly? A Forrester study reveals that data teams spend...
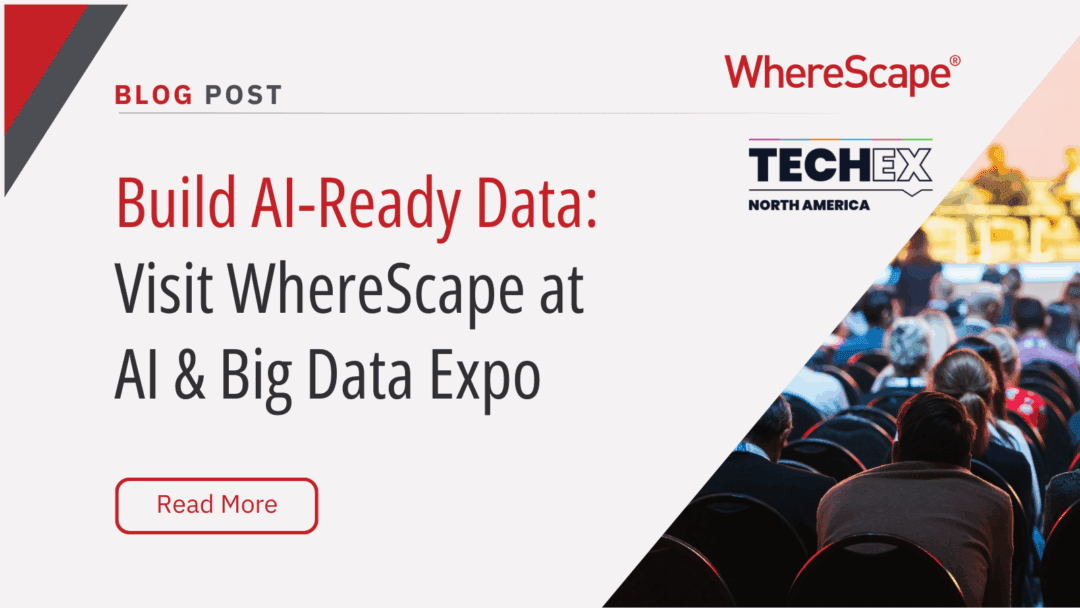
Build AI-Ready Data: Visit WhereScape at AI & Big Data Expo
June 4–5, 2025 | Booth 202 | Santa Clara Convention Center As organizations scale their artificial intelligence and analytics capabilities, the demand for timely, accurate, governed, and AI-ready data has become a strategic priority. According to Gartner, through...
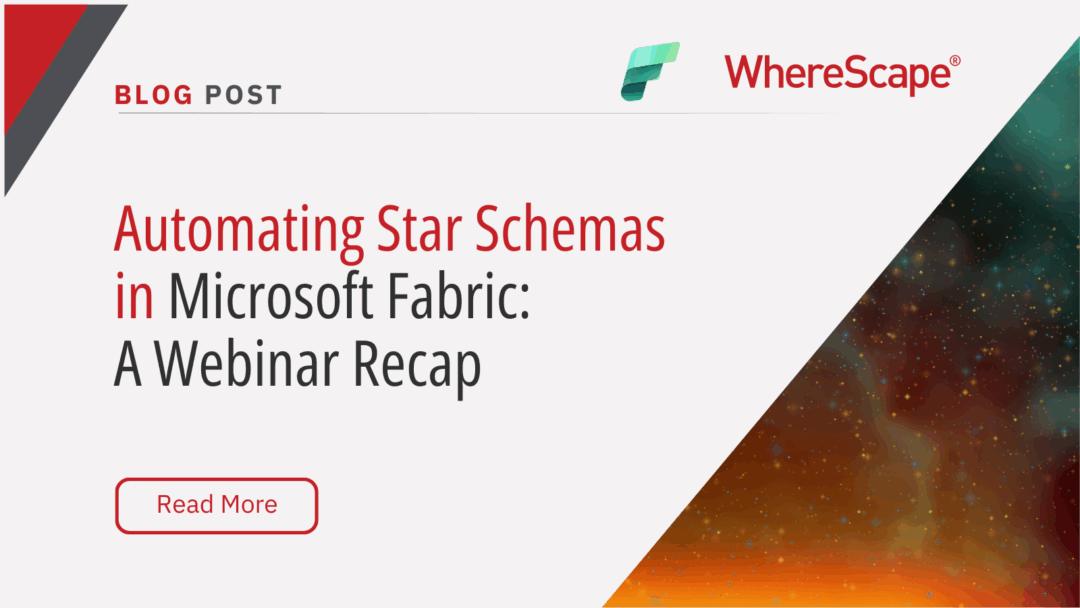
Automating Star Schemas in Microsoft Fabric: A Webinar Recap
From Data Discovery to Deployment—All in One Workflow According to Gartner, data professionals dedicate more than half of their time, 56%, to operational tasks, leaving only 22% for strategic work that drives innovation. This imbalance is especially apparent when...