Ready to explore Data Vault automation...
Data Analytics Platform Migration
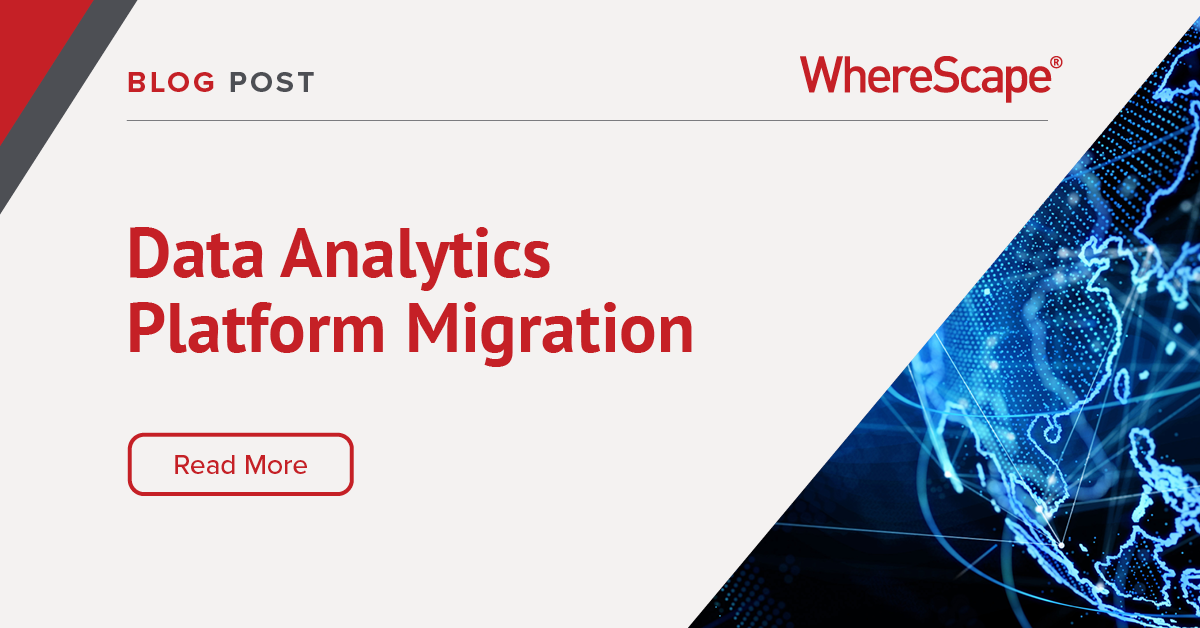
GUEST BLOG POST – Claudia Imhoff, Ph.D.
A thought leader, visionary, and practitioner, Claudia Imhoff, Ph.D., is an internationally recognized expert on analytics, business intelligence, and the architectures to support these initiatives. Dr. Imhoff has co-authored five books on these subjects and writes articles (totaling more than 150) for technical and business magazines.
Migrating to a New Analytics Platform? Here are Some Things to Think About
Many enterprises are considering a move to a new analytics platform, particularly a cloud-based one. Why? Well, there are many reasons – reducing IT costs, reducing data storage costs, improved performance from newer technologies, and many others. But migrating to a new platform is more than just forklifting your legacy data warehouse or data lake into the new environment.
Doing that is a big mistake and a missed opportunity. Aging analytics environments come with several problems such as workarounds that were created to mask problems, production of inefficient code, or projects that valued expediency over good design techniques. Migrating is a great time to blow the dust off the old designs, fix nagging problems, improve overall efficiency of data management processes, remove unused or forgotten data and analytical processes, rationalize all the tools and technologies being used for analysis, and tighten up governance procedures.
ETL
One area that has great potential for improvement is the data transformation or ETL processes. It is this area upon which the remainder of this blog post will focus.
So with this in mind, let’s discuss the technology behind data transformation. ETL or ELT has been around for decades now and yes, you still need mature transformation technology. But for a migration initiative, you need more than just good technology. You need technology that has been created explicitly to ease the migration effort. Ask yourself the following set of questions before embarking on your migration:
- Is your data transformation technology configured to work specifically with whatever cloud platform or platforms you have chosen? Often, enterprises do not settle on a single cloud vendor or a single instance. Make sure your choice of data transformation technology works with and across all the major cloud platforms.
- Does it use the latest cloud platform functionalities and capabilities? Cloud platforms have their own ways of loading and unloading data, as well as other features that must work with the data transformation technology.
- Does it have the proper connectivity for all major sources? Aging analytics environments often have multiple “satellite” sets of analyses occurring outside of the main data warehouse. Migration activities could be used to consolidate some of these disparate environments back into the “mother ship”. These connectors are also used to profile and detect quality problems in the aging environment, as well as browse and discover redundant, unused, or undocumented sets of data.
- What about modern standards for modeling and data transformation procedures? Now would be a good time to enforce standards across the new environment through your data transformation (and other) technologies.
- Does it integrate easily with the other technologies (design, quality, catalog, analysis, etc.) used in an analytics environment? Just enforcing standards from the data model stage through data transformation on to the creation of analytical assets would be a great improvement in many enterprises.
- Does the data management technology have pre-built templates and configurations for different data model types (3NF, Star Schemas, or Data Vault) for all major platforms? It is always faster and better to start from a template than to have to create everything from scratch. Check to make sure your data transformation technology contains patterns as well for specific data modeling styles. Not only are templates and patterns good for standardization but they are terrific productivity tools.
- Can repetitive ETL processes (e.g., dates, times, codes, etc.) be used to relieve some of the tedious programming for the IT staff? These are also great productivity and standardization functions.
- Can you use automation of the data transformation processes to ensure accuracy, timeliness, and up-to-the-minute accuracy of metadata behind these processes? Automation is the key to guaranteeing a successful migration. It is your certification of “goodness” in the final analytical environment.
Cloud Data Migration
Finally, ensure that your data management technology vendor has the data engineers to assist your data architects and data designers in this migration. These resources must be fully capable of delivering and provisioning your new environment on any data platform, for any major cloud configuration. Use these engineers to not only help migrate to the new environment but to fully train your own staff to ultimately replace them.
Once the newly remodeled, redesigned, retransformed analytical environment is up and running, enjoy the benefits of lowered costs, reduced redundancy in data and analytical assets, increased efficiency in data transformations, and improved access to ALL data by the ultimate consumers.
Data Automation
Do you want to know how Data Automation can support your migration to a new analytics platform? Contact WhereScape to find out more.
WhereScape Recap: Highlights From Big Data & AI World London 2025
Big Data & AI World London 2025 brought together thousands of data and AI professionals at ExCeL London—and WhereScape was right in the middle of the action. With automation taking center stage across the industry, it was no surprise that our booth and sessions...
Why WhereScape is the Leading Solution for Healthcare Data Automation
Optimizing Healthcare Data Management with Automation Healthcare organizations manage vast amounts of medical data across EHR systems, billing platforms, clinical research, and operational analytics. However, healthcare data integration remains a challenge due to...
WhereScape Q&A: Your Top Questions Answered on Data Vault and Databricks
During our latest WhereScape webinar, attendees had fantastic questions about Data Vault 2.0, Databricks, and metadata automation. We’ve compiled the best questions and answers to help you understand how WhereScape streamlines data modeling, automation, and...
What is Data Fabric? A Smarter Way for Data Management
As of 2023, the global data fabric market was valued at $2.29 billion and is projected to grow to $12.91 billion by 2032, reflecting the critical role and rapid adoption of data fabric solutions in modern data management. The integration of data fabric solutions...
Want Better AI Data Management? Data Automation is the Answer
Understanding the AI Landscape Imagine losing 6% of your annual revenue—simply due to poor data quality. A recent survey found that underperforming AI models, built using low-quality or inaccurate data, cost companies an average of $406 million annually. Artificial...
RED 10: The ‘Git Friendly’ Revolution for CI/CD in Data Warehousing
For years, WhereScape RED has been the engine that powers rapidly built and high performance data warehouses. And while RED 10 has quietly empowered organizations since its launch in 2023, our latest 10.4 release is a game changer. We have dubbed this landmark update...
The Assembly Line for Your Data: How Automation Transforms Data Projects
Imagine an old-fashioned assembly line. Workers pass components down the line, each adding their own piece. It’s repetitive, prone to errors, and can grind to a halt if one person falls behind. Now, picture the modern version—robots assembling products with speed,...
The Role of Clean Data in AI Success: Avoiding “Garbage In, Garbage Out”
Co-authored by infoVia and WhereScape Artificial Intelligence (AI) is transforming industries across the globe, enabling organizations to uncover insights, automate processes, and make smarter decisions. However, one universal truth remains: the effectiveness of any...
What is a Cloud Data Warehouse?
As organizations increasingly turn to data-driven decision-making, the demand for cloud data warehouses continues to rise. The cloud data warehouse market is projected to grow significantly, reaching $10.42 billion by 2026 with a compound annual growth rate (CAGR) of...
Simplify Cloud Migrations: Webinar Highlights from Mike Ferguson
Migrating your data warehouse to the cloud might feel like navigating uncharted territory, but it doesn’t have to be. In a recent webinar that we recently hosted, Mike Ferguson, CEO of Intelligent Business Strategies, shared actionable insights drawn from his 40+...
Related Content
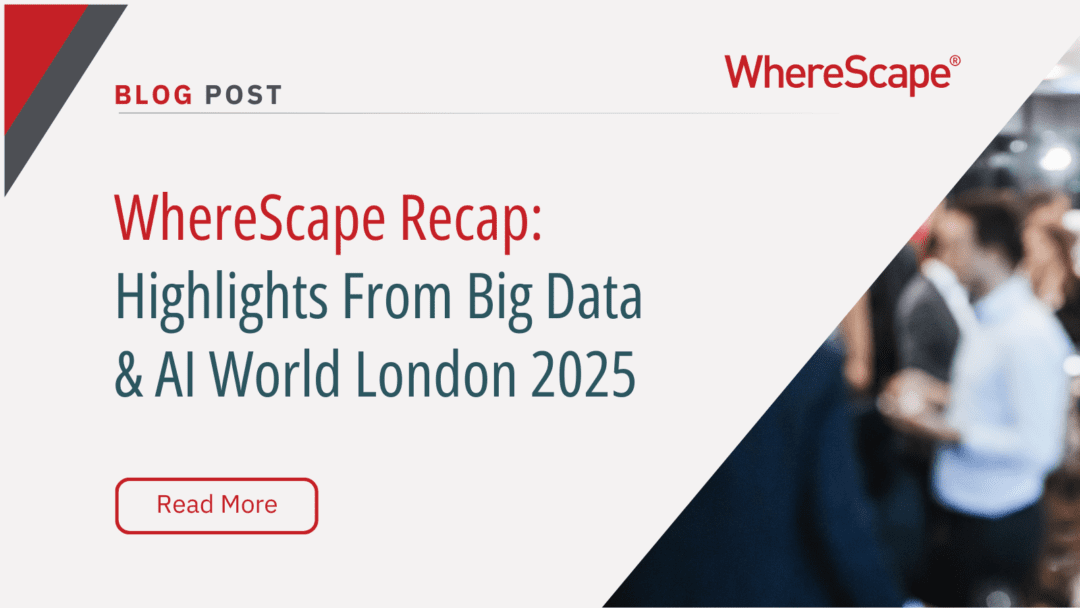
WhereScape Recap: Highlights From Big Data & AI World London 2025
Big Data & AI World London 2025 brought together thousands of data and AI professionals at ExCeL London—and WhereScape was right in the middle of the action. With automation taking center stage across the industry, it was no surprise that our booth and sessions...
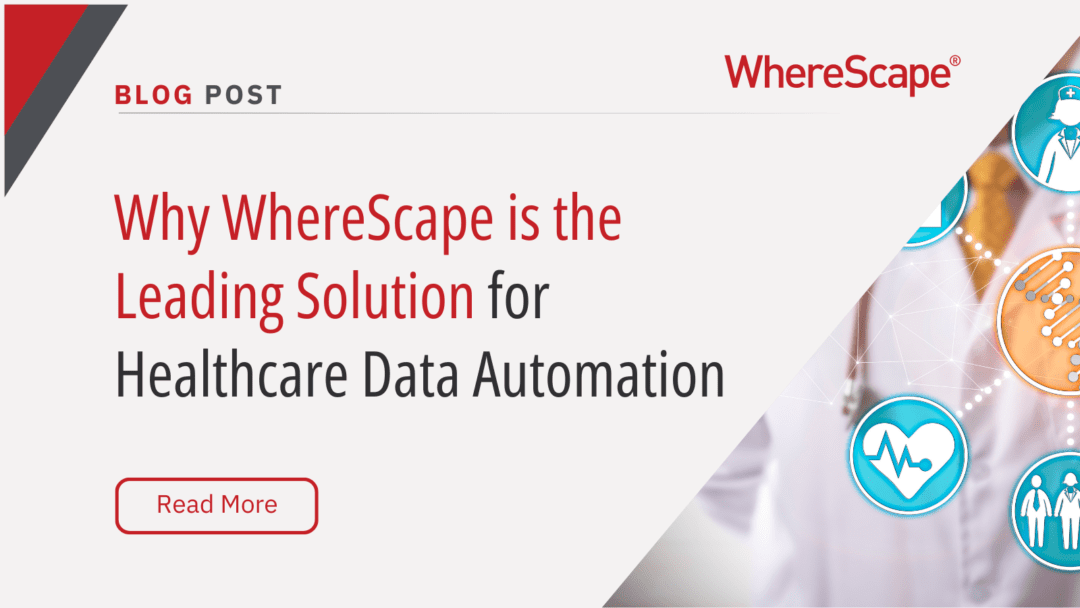
Why WhereScape is the Leading Solution for Healthcare Data Automation
Optimizing Healthcare Data Management with Automation Healthcare organizations manage vast amounts of medical data across EHR systems, billing platforms, clinical research, and operational analytics. However, healthcare data integration remains a challenge due to...
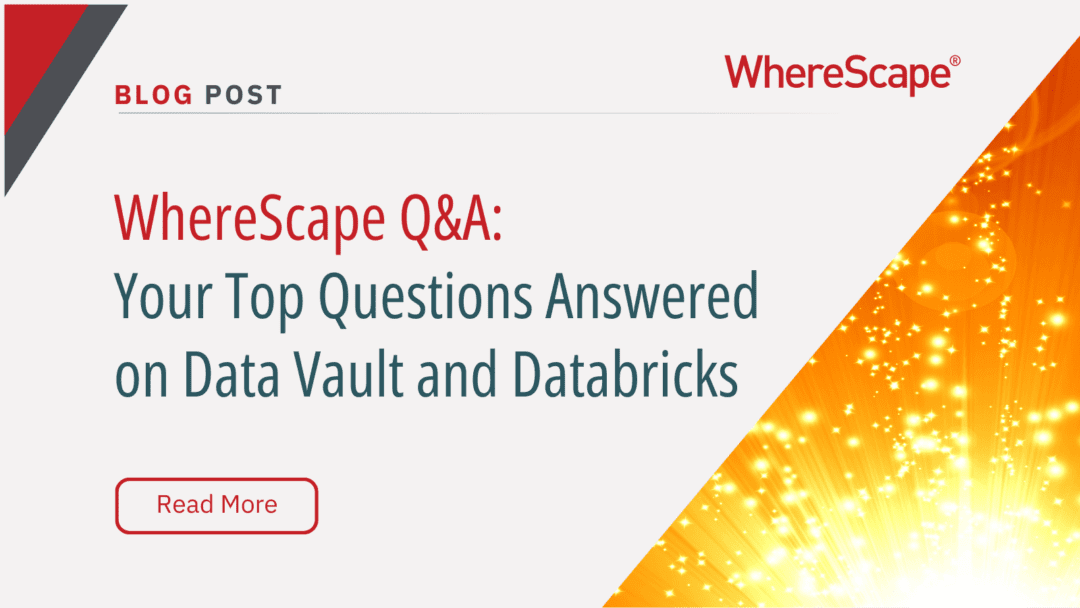
WhereScape Q&A: Your Top Questions Answered on Data Vault and Databricks
During our latest WhereScape webinar, attendees had fantastic questions about Data Vault 2.0, Databricks, and metadata automation. We’ve compiled the best questions and answers to help you understand how WhereScape streamlines data modeling, automation, and...
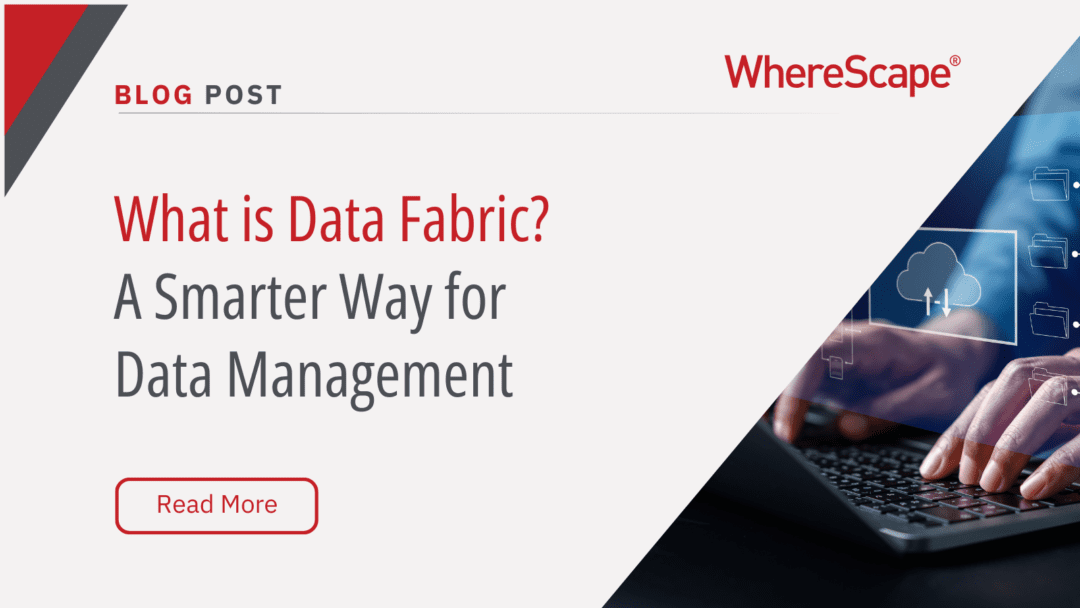
What is Data Fabric? A Smarter Way for Data Management
As of 2023, the global data fabric market was valued at $2.29 billion and is projected to grow to $12.91 billion by 2032, reflecting the critical role and rapid adoption of data fabric solutions in modern data management. The integration of data fabric solutions...