Tune in for a free, live virtual hands-on lab...
Is Data Vault 2.0 Still Relevant?
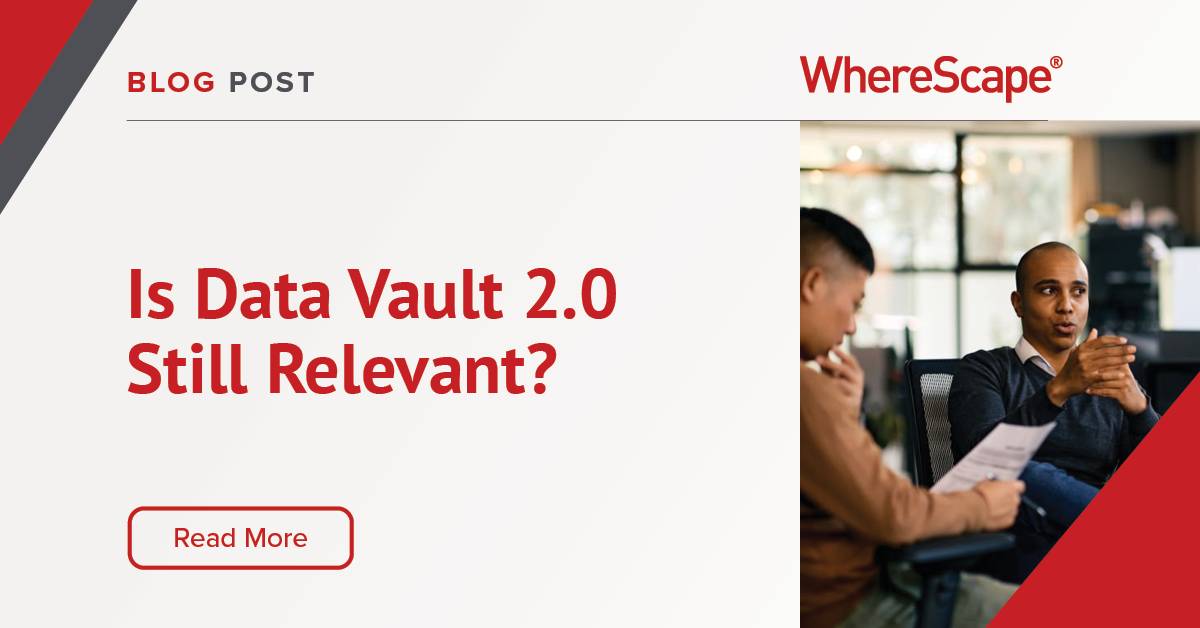
Data Vault 2.0
Data Vault 2.0 is a database modeling method published in 2013. It was designed to overcome many of the shortcomings of data warehouses created using relational modeling (3NF) or star schemas (dimensional modeling). Speci fically, it was designed to be scalable and to handle very large amounts of data. When it was released, a “large” data warehouse may have hundreds of gigabytes or even a terabyte or two. Furthermore, as warehouses become more complex, as more source systems were introduced, the shortcomings of 3NF and star schemas were even more obvious.
Data Vault Scalability
One of the primary design goals of Data Vault 2.0 was to design the data warehouse to be extremely scalable. This is done through two primary characteristics of a data vault. First, the records can be inserted in parallel. With a parent-child relationship, such as order headers and details, the headers usually have to be inserted before the details. Some database engines will not allow you to insert “orphan” records until the parent is loaded. Because of the way Data Vault creates and uses hash keys, every record type can be inserted independently of any other record type. That allows for total parallelism during the load process.
Second, the data vault is designed as a “insert-only” model. With no updates or deletes of records, there will be no transactions or locks on the database during the load process. In addition, there is no database-level referential integrity, so no need to read through primary keys to ensure that relationships are maintained.
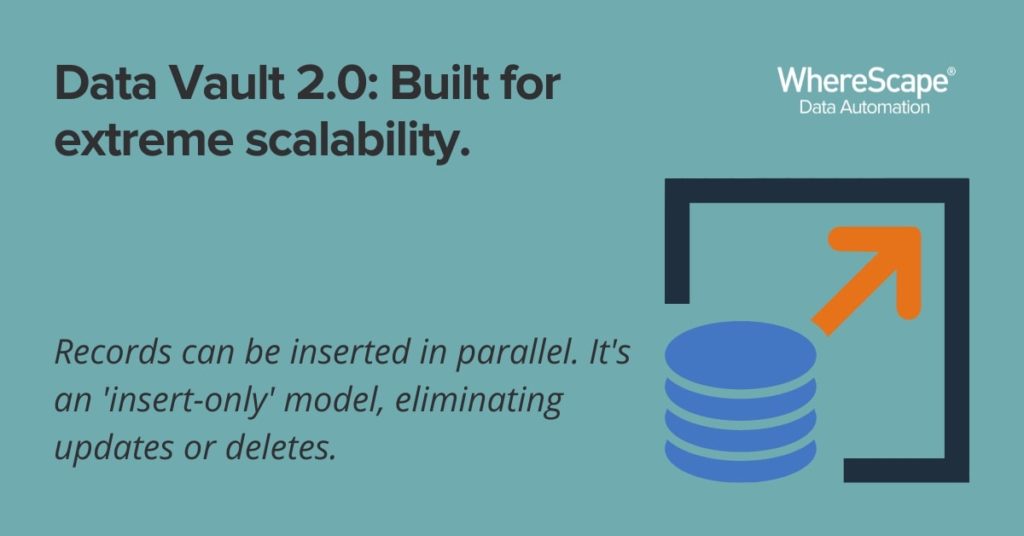
Data Vault 2.0 Methodology
While data warehouses used to be what we would now call a data mart, growth of data, both internally- and externally-generated, along with using more diverse systems as sources, having a data warehouse model that supports quick and easy modification and addition of source systems is critical. The Data Vault 2.0 methodology does this. Adding new source systems, or modifying your model as source systems change, is also handled on an “insert-only” basis. Existing tables and models are not changed, rather new tables and relationships are created. This greatly reduces the scope of testing, as well as reducing the chances of introducing errors.
Data Vault 2.0 Considerations
There are other reasons why Data Vault 2.0 may be the right model for you, such as traceability, working closer with the business, and greater auditability. From a non-technical perspective, these can be as important as the scalability and adaptability of Data Vault 2.0.
Is Data Vault 2.0 Still Relevant?
The Data Vault 2.0 design was released in 2013. It has had several updates now, but the question certainly can be asked: “Is it still relevant?” or “Is there something newer that is better?”
The two greatest factors in making data warehouses has been the exponential (literally) growth of data and source systems. Mergers and acquisitions dictate that disparate systems be integrated into a whole. New data formats (Restful APIs, JSON files, streams, IoT) have all made integration of new data sources critical to the relevancy and lifespan of a data vault. Data Vault 2.0 meets those challenges head-on. For more on WhereScape’s disparate data systems solutions, view this case study.
Data Vault 2.0 is a mature and tested methodology. It has certainly met the challenges of the past and present, and is well positioned to continue to be the major data warehouse design methodology in the foreseeable future. For an enterprise data warehouse, there is no other architecture out there right now that meets the needs of today.
Data Warehouse Automation
Since the initial release of Data Vault 2.0 in 2013, Dan Linstedt, its creator, has said that the single key to success in the design, development, and operation of a data vault has been automation. Warehouse automation software, whether it is for data vault or another methodology, consistently increases productivity, reduces errors, and helps you create a better final product for your data analysts and for your organization. In addition, a data warehouse automation tool, such as WhereScape, allows you to migrate to new targets in the future, whether it is Snowflake, Databricks, Microsoft, or nearly any other data warehouse platform. .
You can check out the many benefits of data warehouse automation for data vault here.
ETL vs ELT: What are the Differences?
In working with hundreds of data teams through WhereScape’s automation platform, we’ve seen this debate evolve as businesses modernize their infrastructure. Each method, ETL vs ELT, offers a unique pathway for transferring raw data into a warehouse, where it can be...
Dimensional Modeling for Machine Learning
Kimball’s dimensional modeling continues to play a critical role in machine learning and data science outcomes, as outlined in the Kimball Group’s 10 Essential Rules of Dimensional Modeling, a framework still widely applied in modern data workflows. In a recent...
Automating Data Vault in Databricks | WhereScape Recap
Automating Data Vault in Databricks can reduce time-to-value by up to 70%—and that’s why we hosted a recent WhereScape webinar to show exactly how. At WhereScape, modern data teams shouldn't have to choose between agility and governance. That's why we hosted a live...
WhereScape Recap: Highlights From Big Data & AI World London 2025
Big Data & AI World London 2025 brought together thousands of data and AI professionals at ExCeL London—and WhereScape was right in the middle of the action. With automation taking center stage across the industry, it was no surprise that our booth and sessions...
Why WhereScape is the Leading Solution for Healthcare Data Automation
Optimizing Healthcare Data Management with Automation Healthcare organizations manage vast amounts of medical data across EHR systems, billing platforms, clinical research, and operational analytics. However, healthcare data integration remains a challenge due to...
WhereScape Q&A: Your Top Questions Answered on Data Vault and Databricks
During our latest WhereScape webinar, attendees had fantastic questions about Data Vault 2.0, Databricks, and metadata automation. We’ve compiled the best questions and answers to help you understand how WhereScape streamlines data modeling, automation, and...
What is Data Fabric? A Smarter Way for Data Management
As of 2023, the global data fabric market was valued at $2.29 billion and is projected to grow to $12.91 billion by 2032, reflecting the critical role and rapid adoption of data fabric solutions in modern data management. The integration of data fabric solutions...
Want Better AI Data Management? Data Automation is the Answer
Understanding the AI Landscape Imagine losing 6% of your annual revenue—simply due to poor data quality. A recent survey found that underperforming AI models, built using low-quality or inaccurate data, cost companies an average of $406 million annually. Artificial...
RED 10: The ‘Git Friendly’ Revolution for CI/CD in Data Warehousing
For years, WhereScape RED has been the engine that powers rapidly built and high performance data warehouses. And while RED 10 has quietly empowered organizations since its launch in 2023, our latest 10.4 release is a game changer. We have dubbed this landmark update...
The Assembly Line for Your Data: How Automation Transforms Data Projects
Imagine an old-fashioned assembly line. Workers pass components down the line, each adding their own piece. It’s repetitive, prone to errors, and can grind to a halt if one person falls behind. Now, picture the modern version—robots assembling products with speed,...
Related Content
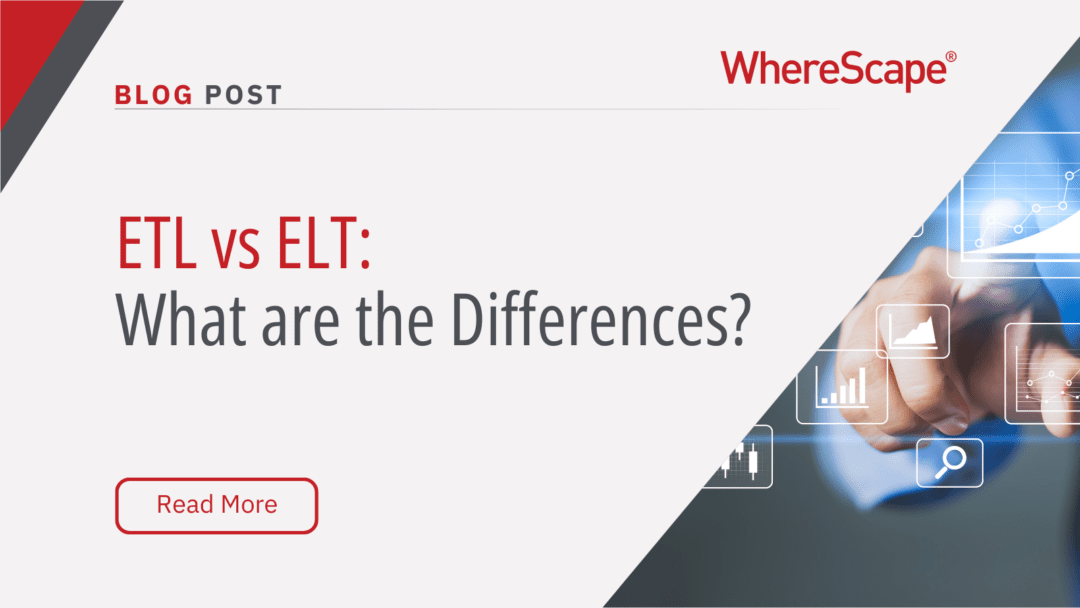
ETL vs ELT: What are the Differences?
In working with hundreds of data teams through WhereScape’s automation platform, we’ve seen this debate evolve as businesses modernize their infrastructure. Each method, ETL vs ELT, offers a unique pathway for transferring raw data into a warehouse, where it can be...
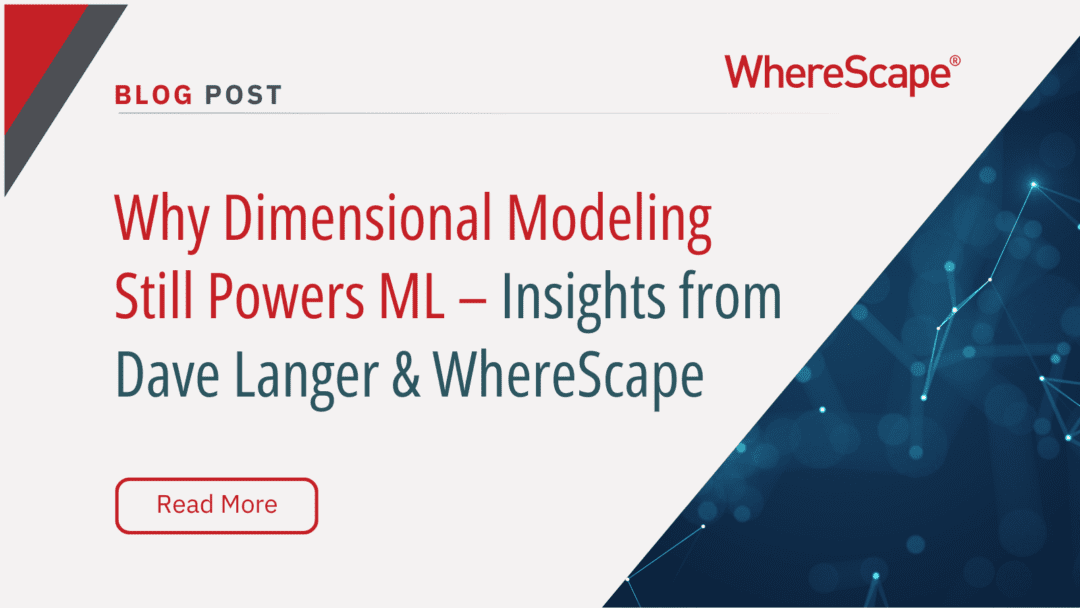
Dimensional Modeling for Machine Learning
Kimball’s dimensional modeling continues to play a critical role in machine learning and data science outcomes, as outlined in the Kimball Group’s 10 Essential Rules of Dimensional Modeling, a framework still widely applied in modern data workflows. In a recent...
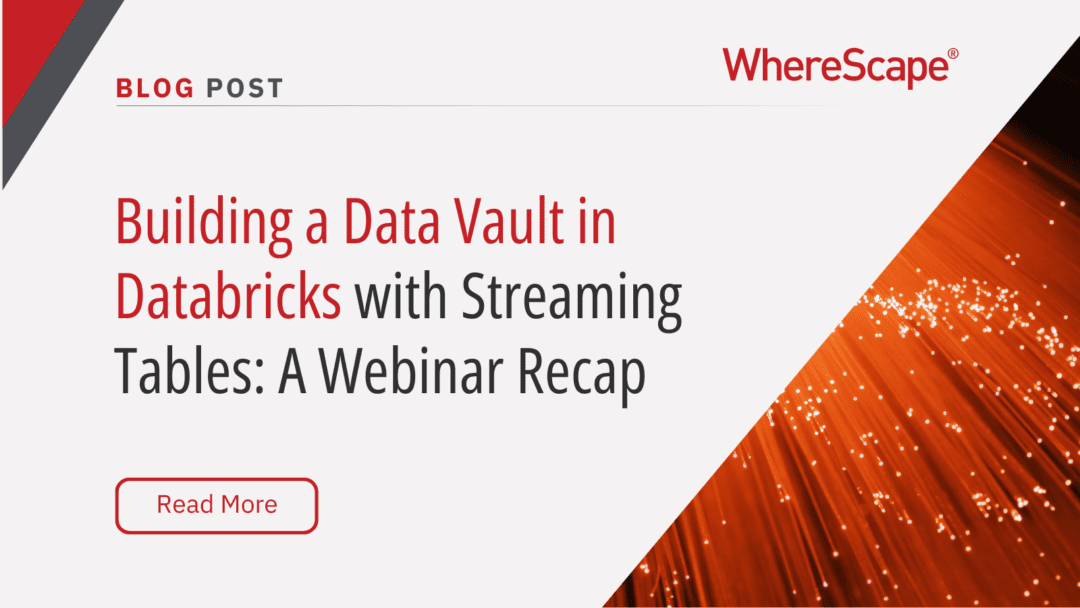
Automating Data Vault in Databricks | WhereScape Recap
Automating Data Vault in Databricks can reduce time-to-value by up to 70%—and that’s why we hosted a recent WhereScape webinar to show exactly how. At WhereScape, modern data teams shouldn't have to choose between agility and governance. That's why we hosted a live...
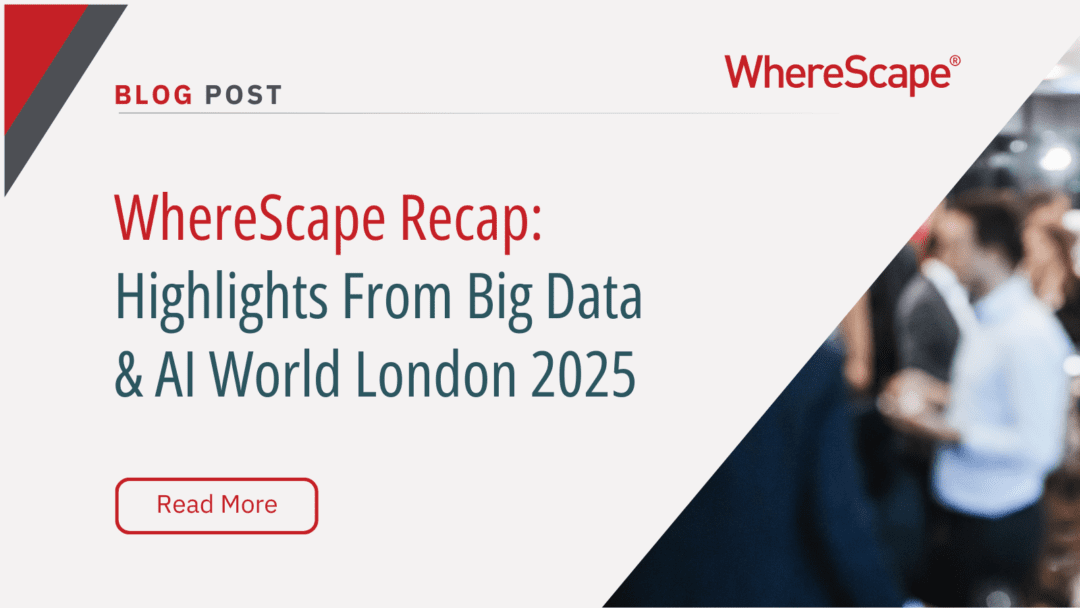
WhereScape Recap: Highlights From Big Data & AI World London 2025
Big Data & AI World London 2025 brought together thousands of data and AI professionals at ExCeL London—and WhereScape was right in the middle of the action. With automation taking center stage across the industry, it was no surprise that our booth and sessions...