Join us for an insightful webinar where we...
Data Automation Levels
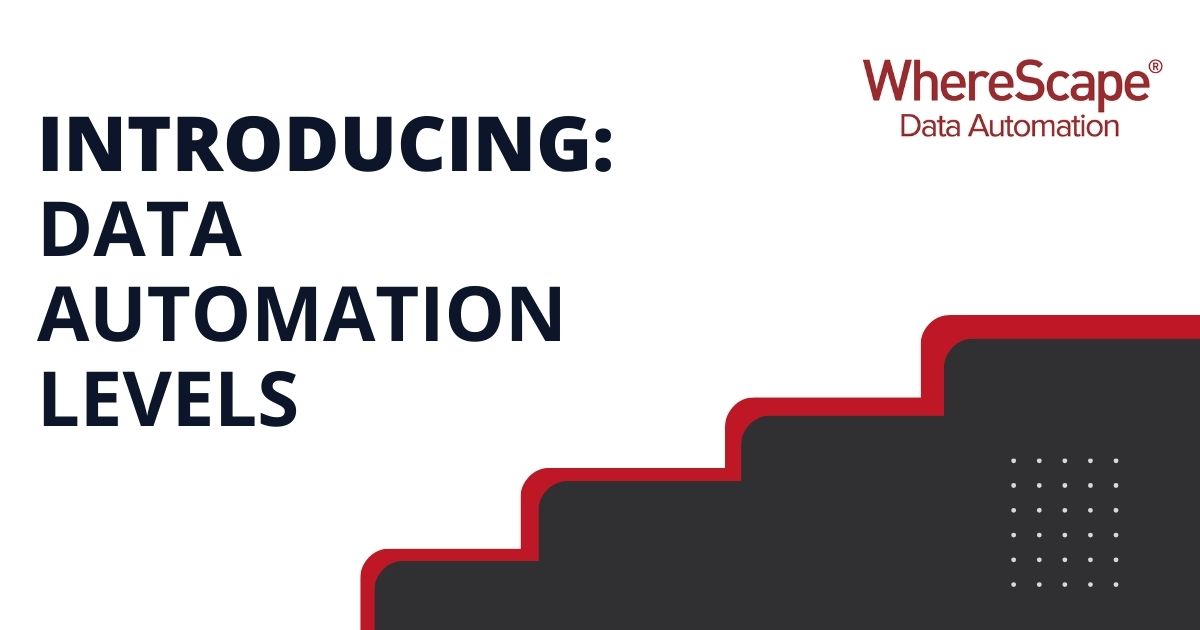
The concept of automation has seamlessly integrated into many aspects of our lives, from self-driving cars to sophisticated software systems. Recently, Mercedes-Benz announced their achievement in reaching Level 3 in automated driving technology, which got me thinking about the parallels in the world of Data Warehouse Automation (DWA).
Just as in the realm of autonomous vehicles, DWA isn’t a binary state but rather exists on a spectrum of capabilities. In this article, I’ll explore the various levels of DWA, demystifying how they function and what each level means for businesses and technologists alike. This journey through the layers of automation will provide insights into not only how DWA is evolving but also its potential impact on our data-driven future.
Data Warehouse Automation Levels: From Cars to Coding
The United States National Highway Transportation Safety Administration has defined six levels of automated driving, primarily differentiated by the degree of driver involvement. This gradation of automation ranges from no automation (Level 0) where the driver performs all tasks, to full automation (Level 5), where no driver is needed at all.
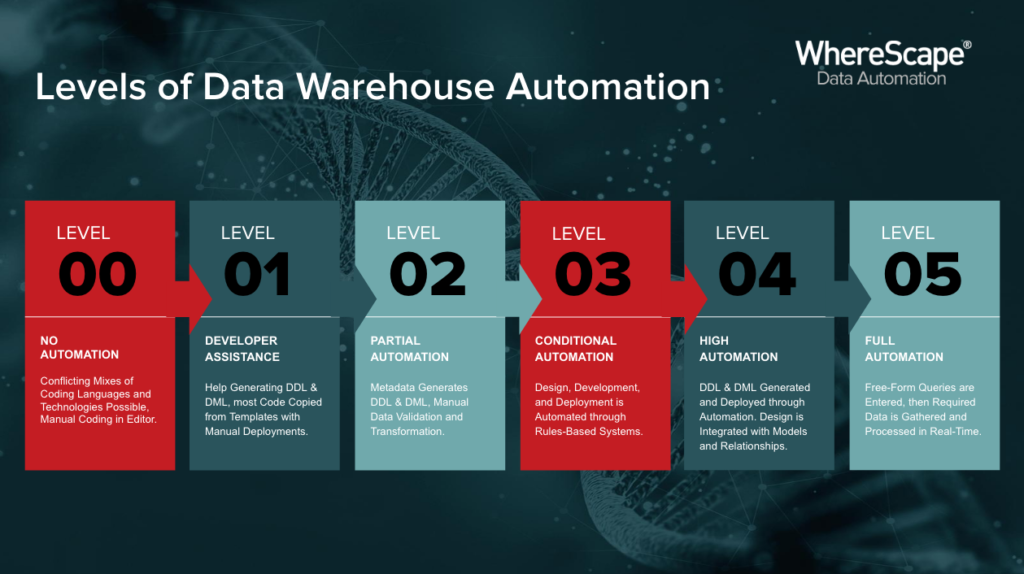
This concept of varying levels of automation isn’t limited to the world of vehicular transport. It extends into my daily work realm – Data Warehouse Automation (DWA). Similar to the automotive industry, DWA encompasses a spectrum of capabilities. These capabilities can be categorized into levels, much like the automated driving system. Though not formally recognized, if we were to define levels 0-5 for DWA, they would represent a progression from manual data handling and analysis (Level 0) to a fully autonomous data management system (Level 5) where human intervention is minimal or unnecessary.
This gradation hints at an evolving landscape in data management, where efficiency, accuracy, and speed are continually enhanced through increasing levels of automation. I hadn’t thought about it formally, but if we had levels of 0-5 for DWA, what would they be?
Level 0 – No Automation
Everything is done manually. Design work is done on paper, or with minimal tools. Requirements and data profiling are done manually. Development of the structures and pipelines for storing and moving data are written by hand and then executed in a database administrative tool.
Level 1 – Developer Assistance
An entity-relationship tool is used to create the logical model of the data warehouse. Templates are used to write the DDL and DML code. Deployment and documentation are still manual processes.
Level 2 – Partial Automation
DDL and DML are generated from metadata, but no knowledge of data warehouse design or relationships. The code is manually deployed. Any data validation or transformation is done manually. Deep technical knowledge and experience is required.
Level 3 – Conditional Automation
Design, development, and deployment are created with an understanding of data warehouse architecture. Rules-based systems automatically apply attributes and transform data as needed. Profiling, design, creation of ELT processes, and linking various object types (such as facts and dimensions) are all done automatically. Deployments, documentation, and task scheduling are all handled automatically.
Level 4 – High Automation
DDL and DML generated and deployed. Design is fully automated, and relationships and data warehouse models are implemented automatically through data catalogs. Very, very little code is hand-written. All of the development and deployment of the data warehouse is managed by analysts, rather than technical staff.
Level 5 – Full Automation
Free-form queries are entered and the required data is gathered from multiple sources and processed in real-time. Does the data warehouse even exist anymore? Artificial intelligence might be involved in caching and calculating data before it is needed, but the idea of a dedicated online analytics system with batch jobs loading data for later analysis has been rendered obsolete. Why do I need a data warehouse if I can simply get the answer to any question I ask?
Why Automated Driving?
With automated driving, the first reaction of most people is “Great, I can relax while the car drives itself.” But fully automated driving is going to drastically change how we use cars, probably in ways we can’t predict. Some benefits would be faster commutes, less congestion meaning more sustainability, better use of intersections, fewer accidents, lower maintenance costs, greatly increased gas mileage, lower insurance premiums, and so on. Perhaps even the private ownership of cars may go away, and they will simply be available on demand.
Why Data Warehouse Automation?
Faster building of a data warehouse is a key benefit of DWA. But, as with driving, there are unexpected benefits:
Data Automation for Productivity
WhereScape RED transforms the development landscape with its drag-and-drop approach, significantly shortening the time needed for data infrastructure development, deployment, and operations. This automation leads to a streamlined workflow that not only saves time but also ensures consistency across projects.
Platform-Native Code Generation
One of the most striking features of WhereScape RED is its ability to eliminate up to 95% of manual coding. By automatically generating SQL and other codes native to your target platform, it adheres to platform-specific best practices, boosting productivity and reducing the risk of inconsistencies.
Automatic Documentation and Metadata Management
Keeping documentation updated is a cumbersome task, but with WhereScape RED, this happens automatically. The tool not only maintains comprehensive documentation but also manages metadata efficiently. This feature ensures an up-to-date, transparent view of your data infrastructure, essential for both IT and business stakeholders.
Agile Data Warehouse Development
WhereScape RED is embedded with best practices for various data warehousing methodologies like 3NF, Data Vault, and dimensional modeling. This integration reduces complexity and accelerates development. Furthermore, its integrated scheduling and workflow engine simplifies the management of decision support infrastructure, eliminating the need for manual scripting.
Advanced SQL Code Generation
The tool excels in generating native SQL code, leveraging database-specific features and applications. Additionally, it automates the entire data warehousing life cycle, from design to operation, with its integrated metadata repository and support for agile methodologies.
Agile Prototyping
WhereScape RED empowers users to move swiftly from source data to a populated schema, facilitating rapid prototyping. It also excels in integrating big data infrastructure, such as data lakes, with enterprise data, thus creating a comprehensive understanding across the business landscape.
ELT and Data Lineage
Offering complete extraction, load, and transformation capabilities, WhereScape RED includes integrated dependency management and scheduling. Its data lineage visualization aids in understanding the flow of data and the impact of changes, a crucial aspect of modern data management.
Data Automation Today
There are several companies offering data warehouse automation tools today. WhereScape 3D and WhereScape RED, probably the most advanced tools, are around Level 3. With the adoption of data fabric and more advanced data cataloging, I expect Level 4 automation to come about in the next three to five years. Full automation? I think you’re going to find that’s going to be much easier in a car than in an open technical environment that requires data analytics.
Data Warehouse Automation is a necessity. If you are looking at DWA tools, think about how advanced each tool is. How flexible is it? How does it work in my current (or future) technical stack? How abstractly am I working? Am I telling the tool WHAT I want to do or HOW I want to do it? A good tool will know the HOW. You should simply provide the WHAT.
Embracing Data Automation in Data Warehousing
Data Warehouse Automation is more than a trend. It’s a significant shift in data handling and processing. The progression from Level 0 to Level 5 in data warehouse automation reflects our move towards a more automated and intelligent future. This evolution brings substantial benefits of data warehouse automation, including reduced costs, enhanced data processing speed and accuracy, and a transformative approach to business decision-making.
While reaching the pinnacle of Level 5 in DWA presents its challenges, it opens up a world of possibilities in data management. For businesses, adapting to these changes and choosing the right tools, like WhereScape’s offerings, are crucial steps in leveraging the potential of DWA. Let’s move forward into this automated future, recognizing that the journey is as much about visionary thinking as it is about technological advancement.
Ready to see how WhereScape can revolutionize your data strategy? Book a demo today and take the first step towards a smarter, more efficient data future.
Experience the Power of WhereScape 3D 9.0.3: New Features and Improvements
We’re thrilled to introduce our latest iteration of WhereScape 3D! Version 9.0.3 brings a host of new features and enhancements designed to make your data warehousing journey smoother, faster, and more efficient. Let’s dive into the details of what you can expect from...
Ahead of the Curve: Future Trends in Data Automation and WhereScape’s Pioneering Solutions
The Evolving Landscape of Data Automation As new technologies emerge and existing tools constantly change and improve, the world of data automation transforms rapidly. Even the most well-versed data teams find themselves disoriented and overwhelmed in the face of...
Investing in Data Automation: A Strategic Approach to Business Growth
Unlocking Growth: The Strategic Advantage of Data Automation Organizations reaping the benefits of data automation stay ahead of industry trends and improve the efficiency of their operations and decision-making. Data automation tools offer a strategic advantage for...
Data + AI Summit 2024: Key Takeaways and Innovations
The Data + AI Summit 2024, hosted by Databricks at the bustling Moscone Center in San Francisco, has concluded with remarkable revelations and forward-looking innovations. Drawing over 16,000 attendees in person and virtually connecting over 60,000 participants from...
WhereScape RED 10.1 is Here: Enhanced Scheduling and Customization
We’re proud to announce the highly anticipated WhereScape RED 10.1 is now available, and it’s packed with exciting new features and enhancements designed to make your data warehousing experience more efficient and enjoyable. Let's take a closer look at what’s new and...
Supercharging Data Integration: The WhereScape and Databricks Advantage
The demand for robust data management systems has never been higher, and Databricks has quickly become a favored choice for cloud-based solutions. Its powerful capabilities make it a top contender for managing large-scale data, but when combined with WhereScape's...
Empowering Customer Success: WhereScape’s Comprehensive Support and Training Resources
Enhancing Operational Success with WhereScape’s Support Systems At WhereScape, we understand that a data warehouse is only useful to the extent that it is understood. In order to drive your organization closer to your key goals and objectives, you need full mastery of...
Revolutionizing Day-to-Day Operations: The Power of Automated Data Integration
The Transformational Role of Automation in Data Management Across industries and business stages, organizations of all types manage data in their daily operations. Whether that data entails patient appointments and reminders in a healthcare clinic, student performance...
Gartner® Insights: Microsoft Fabric as a Unified Data & Analytics Platform
Are you ready to revolutionize your data management strategy with a platform that promises to simplify and enhance your operations? According to a Gartner poll, 43% of respondents believe that the data and analytics ecosystem will significantly influence their choice...
WhereScape and YellowFin Attending World of Data in Munich
We are excited to announce that WhereScape and YellowFin will be attending the World of Data conference in Munich on June 6, 2024. This event will bring together data professionals, industry leaders, and technology enthusiasts from around the globe to explore the...
Related Content
Experience the Power of WhereScape 3D 9.0.3: New Features and Improvements
We’re thrilled to introduce our latest iteration of WhereScape 3D! Version 9.0.3 brings a host of new features and enhancements designed to make your data warehousing journey smoother, faster, and more efficient. Let’s dive into the details of what you can expect from...
Ahead of the Curve: Future Trends in Data Automation and WhereScape’s Pioneering Solutions
The Evolving Landscape of Data Automation As new technologies emerge and existing tools constantly change and improve, the world of data automation transforms rapidly. Even the most well-versed data teams find themselves disoriented and overwhelmed in the face of...
Investing in Data Automation: A Strategic Approach to Business Growth
Unlocking Growth: The Strategic Advantage of Data Automation Organizations reaping the benefits of data automation stay ahead of industry trends and improve the efficiency of their operations and decision-making. Data automation tools offer a strategic advantage for...
Data + AI Summit 2024: Key Takeaways and Innovations
The Data + AI Summit 2024, hosted by Databricks at the bustling Moscone Center in San Francisco, has concluded with remarkable revelations and forward-looking innovations. Drawing over 16,000 attendees in person and virtually connecting over 60,000 participants from...