Tune in for a free, live virtual hands-on lab...
Guide to Data Quality: Ensuring Accuracy and Consistency in Your Organization
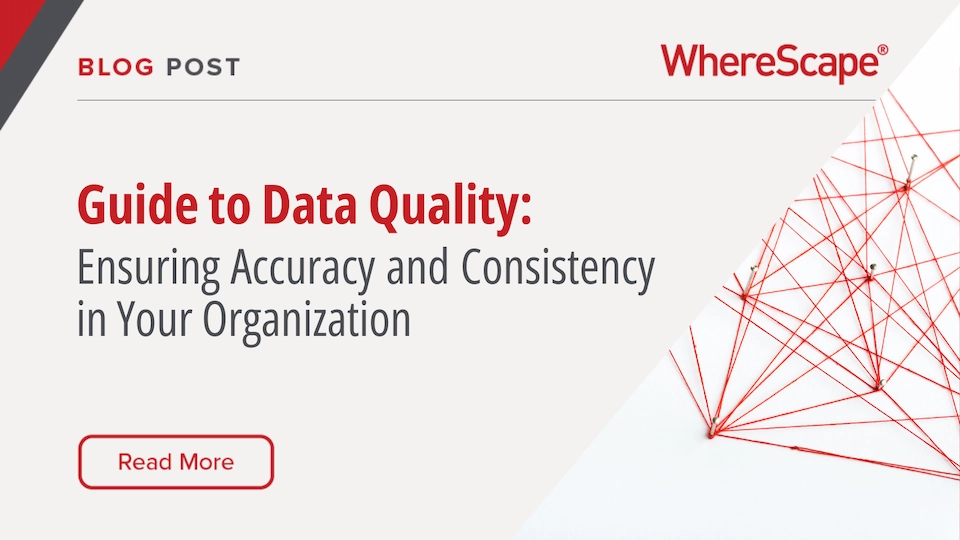
Why Data Quality Matters
Data is only as useful as it is accurate and complete. No matter how many analysis models and data review routines you put into place, your organization can’t truly make data-driven decisions without accurate, relevant, complete, and consistent data.
What’s more, low-quality data leads to mix-ups and errors that stain your customer service record and can even result in legal and regulatory issues. Tending to data quality standards is a top priority for ensuring efficient business functions, analytical decision-making, and accurate workflows.
Understanding Data Quality
Data quality consists of multiple components that all play into the overall usefulness and reliability of a group of data. Understanding the key factors of data quality can help you make an action plan for setting and maintaining high data quality standards.
What Is Data Quality?
Data quality describes how accurate, complete, consistent, and valid a dataset is. In order to make confident decisions based on data, leaders need to know that their data is reliable and accurate. Low data quality standards lead to business risks, inefficiencies, and costly mistakes.
Key Components of Data Quality
To determine data quality, teams must consider data accuracy, data consistency, and data integrity. It’s also important to keep the end use in mind to ensure that the specific data points are relevant to the exact problem you’re trying to solve.
Data Accuracy
Data accuracy measures whether data reflects reality. Errors like typos and outdated information all reduce the accuracy of a dataset. High data accuracy requires minimal or no errors. Working toward data accuracy ensures that an organization can make strategic data-based decisions.
Data Consistency
In addition to being accurate, useful data is also consistent. Data consistency requires uniform, coherent data across platforms and storage locations. To achieve data consistency, teams must align formatting and definitions to avoid discrepancies that decrease the usefulness of the data. Through seamless and intentional integration processes, using tools like WhereScape 3D, teams can maintain data consistency and enhance overall trust in data.
Data Integrity
Data integrity refers to the completeness and reliability of data over time. Throughout the entire data lifecycle, actions like transferring, integrating, and reformatting data can cause unintentional changes to datasets. When a dataset loses its integrity, it’s no longer comprehensive and may become less useful for its intended purpose.
Best Practices for Ensuring High Data Quality
Armed with high-quality data, organizations make advanced decisions to benefit the present and future of the business. To ensure high data quality standards, organizations can prioritize the following three elements of data handling.
Establishing Data Quality Standards
The primary factor in ensuring high data quality is establishing ideal data quality standards. Data quality standards should outline clear, measurable criteria describing exactly what constitutes high-quality data for your specific organization’s goals. In order to establish these standards, set benchmarks for the accuracy, consistency, integrity, timeliness, and validity of reliable and useful data.
With these data quality standards clearly defined, organizations can operate with a shared understanding of expectations and maintain uniform data collection, processing, and analysis practices. In order to establish data quality standards, organizations can follow these key steps:
- Start by reviewing current processes to identify gaps and shortcomings
- Establish regular monitoring routines
- Train all staff on best practices.
These steps foster a culture of accountability and help everyone play a role in and benefit from high data quality standards.
Data Governance and Management
Data governance and management describe the policies and processes that ensure your data assets remain reliable and organized over time. Data governance requires well-defined ownership roles and a strong sense of accountability throughout the organization. With reliable rules and procedures for how data is collected, stored, accessed, and shared, organizations can more easily align data practices with business objectives. These steps also ensure regulatory compliance, reducing risks of legal ramifications.
Data management complements governance with a focus on the practical aspects of handling data. Aspects of data management include:
- Storage
- Retrieval
- Integration
- Maintenance
Leveraged together, with careful data governance and management using WhereScape’s automation software, businesses create a structured approach that mitigates risks related to data breaches and inaccuracies, ensuring data quality remains high..
Data Validation and Cleaning
Data validation and cleaning are crucial to keeping a high level of data accuracy and overall data quality. Data validation requires teams to systematically check data against predefined rules and criteria in order to make sure it meets data standards before use in decision-making or analysis.
Completing frequent data validation processes helps organizations catch errors early on in the data lifecycle before they’ve had a chance to negatively impact data-driven decisions. Data validation includes:
- Verifying data types
- Checking data formats
- Matching data ranges
- Confirming the presence of required fields
On the other side of the same coin, data cleaning requires teams to remove incorrect, incomplete, and duplicated data from a database. This process improves data quality and data consistency. Data cleaning activities include:
- Standardizing data formats
- Filling in missing values
- Resolving inconsistencies
Establishing a predictable and routine cadence for both data validation and cleaning is essential for maintaining high data quality standards. Tools like WhereScape Data Vault Express can significantly streamline these processes. Since data evolves nonstop, new errors arise and it’s important to catch them before they impact future reports.
Implementing a Data Quality Strategy
Combine the best practices above into a comprehensive data quality strategy that fits your workflows, workforce, and business priorities.
Steps to Develop a Data Quality Plan
To develop a thorough data quality plan that addresses the unique needs of your business, follow these steps:
- Meet with all stakeholders within your organization. Stakeholders include anyone who leads a team that uses, organizes, manages, cleans, or inputs data. Communicate about goals and role assignments for your ongoing data quality improvement plan.
- Set measurable goals using well-defined KPIs and predefined monitoring cadences.
- Identify your organization’s core data elements and tools.
- Assess your current data assets to determine data quality and your main data quality challenges.
- Outline areas for improvement and work together to determine plans for increasing data quality.
- Conduct regular audits and monitor accuracy, consistency, and integrity of data on an ongoing basis.
- Use advanced data management tools like WhereScape to reduce errors and save team member time.
- Foster a data-driven culture where every team member understands and plays a role in maintaining data quality.
The Path to Better Data Quality
The long road to improving data quality can feel overwhelming, and without the proper tools, it can cause strain on an organization’s workflows and operations. However, with the right tools on your side, the path to better data quality is paved with ease and efficiency. WhereScape helps boost productivity, reduce errors, and multiply ROI through advanced and accurate data-driven decision-making.
Book a demo to see how WhereScape can transform your data quality and your business.
ETL vs ELT: What are the Differences?
In working with hundreds of data teams through WhereScape’s automation platform, we’ve seen this debate evolve as businesses modernize their infrastructure. Each method, ETL vs ELT, offers a unique pathway for transferring raw data into a warehouse, where it can be...
Dimensional Modeling for Machine Learning
Kimball’s dimensional modeling continues to play a critical role in machine learning and data science outcomes, as outlined in the Kimball Group’s 10 Essential Rules of Dimensional Modeling, a framework still widely applied in modern data workflows. In a recent...
Automating Data Vault in Databricks | WhereScape Recap
Automating Data Vault in Databricks can reduce time-to-value by up to 70%—and that’s why we hosted a recent WhereScape webinar to show exactly how. At WhereScape, modern data teams shouldn't have to choose between agility and governance. That's why we hosted a live...
WhereScape Recap: Highlights From Big Data & AI World London 2025
Big Data & AI World London 2025 brought together thousands of data and AI professionals at ExCeL London—and WhereScape was right in the middle of the action. With automation taking center stage across the industry, it was no surprise that our booth and sessions...
Why WhereScape is the Leading Solution for Healthcare Data Automation
Optimizing Healthcare Data Management with Automation Healthcare organizations manage vast amounts of medical data across EHR systems, billing platforms, clinical research, and operational analytics. However, healthcare data integration remains a challenge due to...
WhereScape Q&A: Your Top Questions Answered on Data Vault and Databricks
During our latest WhereScape webinar, attendees had fantastic questions about Data Vault 2.0, Databricks, and metadata automation. We’ve compiled the best questions and answers to help you understand how WhereScape streamlines data modeling, automation, and...
What is Data Fabric? A Smarter Way for Data Management
As of 2023, the global data fabric market was valued at $2.29 billion and is projected to grow to $12.91 billion by 2032, reflecting the critical role and rapid adoption of data fabric solutions in modern data management. The integration of data fabric solutions...
Want Better AI Data Management? Data Automation is the Answer
Understanding the AI Landscape Imagine losing 6% of your annual revenue—simply due to poor data quality. A recent survey found that underperforming AI models, built using low-quality or inaccurate data, cost companies an average of $406 million annually. Artificial...
RED 10: The ‘Git Friendly’ Revolution for CI/CD in Data Warehousing
For years, WhereScape RED has been the engine that powers rapidly built and high performance data warehouses. And while RED 10 has quietly empowered organizations since its launch in 2023, our latest 10.4 release is a game changer. We have dubbed this landmark update...
The Assembly Line for Your Data: How Automation Transforms Data Projects
Imagine an old-fashioned assembly line. Workers pass components down the line, each adding their own piece. It’s repetitive, prone to errors, and can grind to a halt if one person falls behind. Now, picture the modern version—robots assembling products with speed,...
Related Content
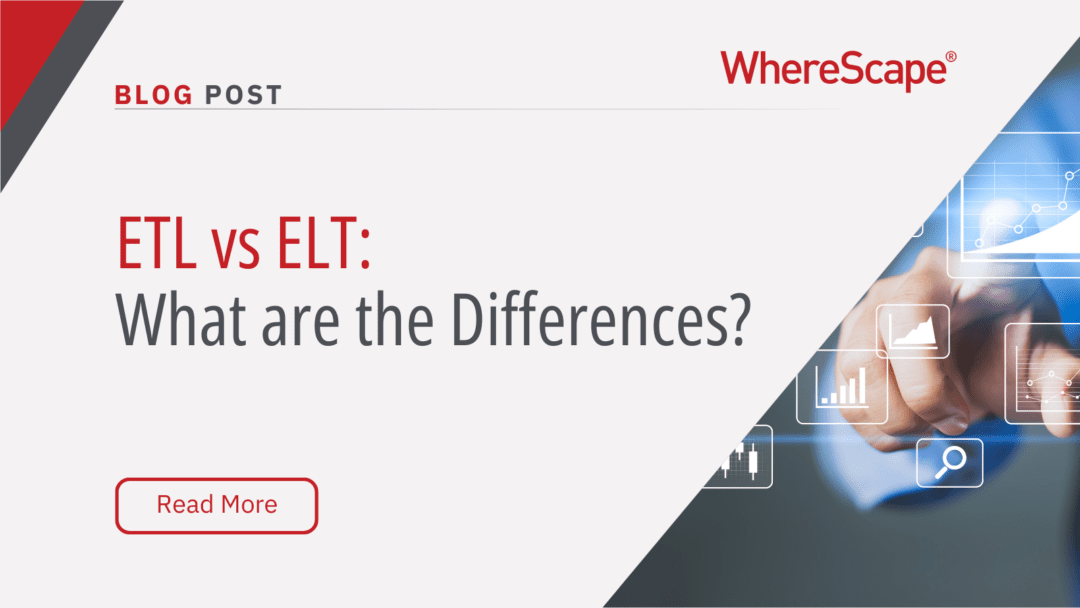
ETL vs ELT: What are the Differences?
In working with hundreds of data teams through WhereScape’s automation platform, we’ve seen this debate evolve as businesses modernize their infrastructure. Each method, ETL vs ELT, offers a unique pathway for transferring raw data into a warehouse, where it can be...
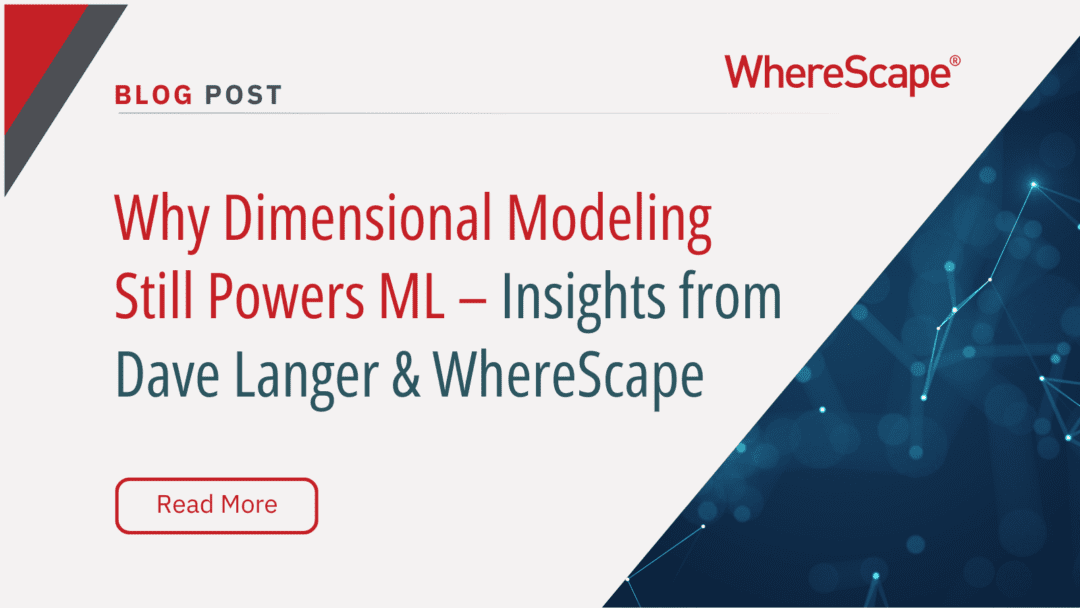
Dimensional Modeling for Machine Learning
Kimball’s dimensional modeling continues to play a critical role in machine learning and data science outcomes, as outlined in the Kimball Group’s 10 Essential Rules of Dimensional Modeling, a framework still widely applied in modern data workflows. In a recent...
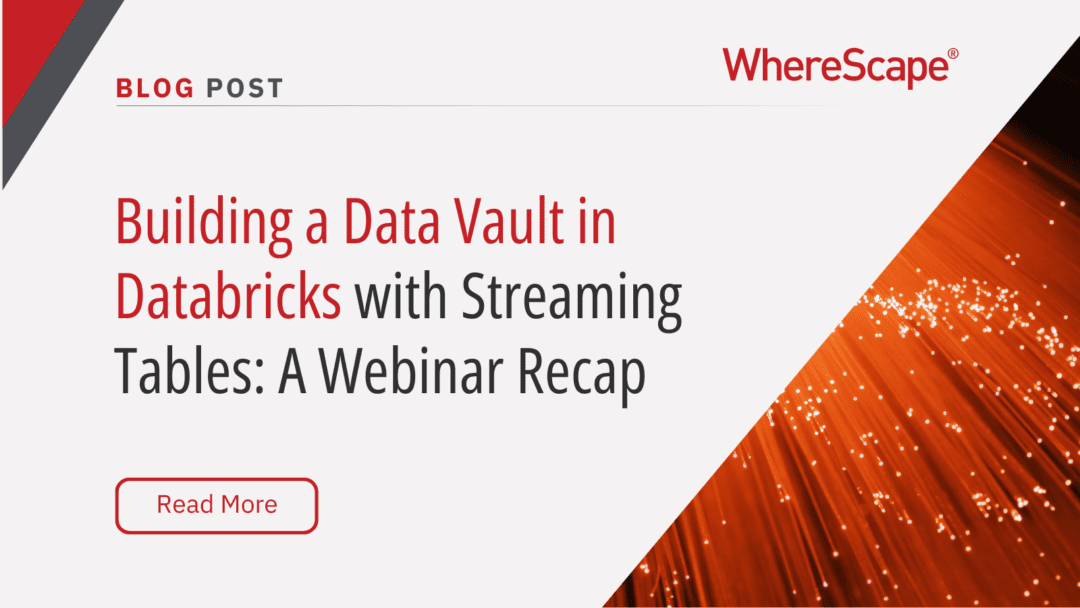
Automating Data Vault in Databricks | WhereScape Recap
Automating Data Vault in Databricks can reduce time-to-value by up to 70%—and that’s why we hosted a recent WhereScape webinar to show exactly how. At WhereScape, modern data teams shouldn't have to choose between agility and governance. That's why we hosted a live...
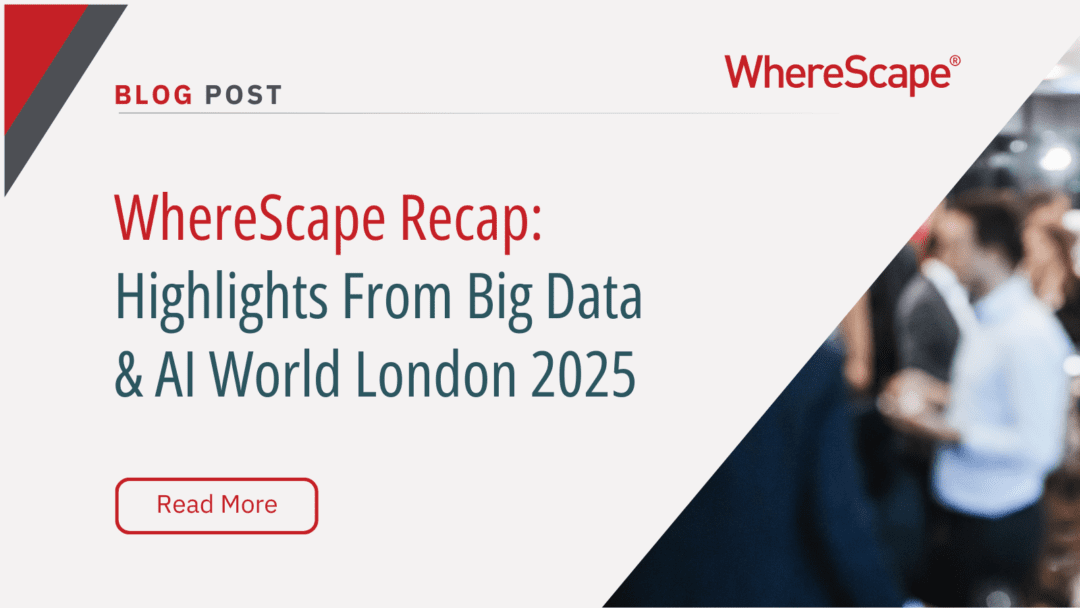
WhereScape Recap: Highlights From Big Data & AI World London 2025
Big Data & AI World London 2025 brought together thousands of data and AI professionals at ExCeL London—and WhereScape was right in the middle of the action. With automation taking center stage across the industry, it was no surprise that our booth and sessions...