Join our expert-led virtual hands-on lab and...
A Webinar Recap: Exploring Data Automation Levels with Kent Graziano
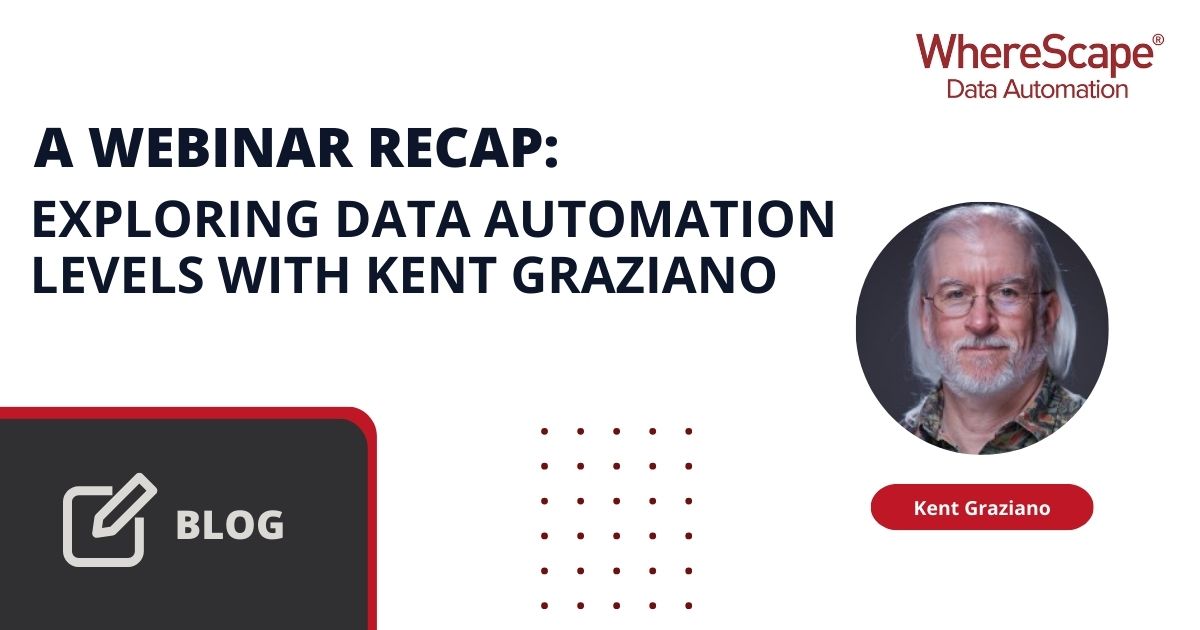
Our most recent webinar, “The Future of Data Warehousing: Understanding Automation Levels,” hosted by Patrick O’Halloran, Solutions Architect, and esteemed guest speaker Kent Graziano dove into the transformative world of data warehouse automation. They discussed its impacts, the levels, and the evolution of automation in the field, and speculated about future developments.
The Evolution of Automation in Data Warehousing
The development of data warehouses has always been complex and vulnerable to financial risks. However, with the advent of automation technologies, these challenges are increasingly being mitigated. Patrick began by tracing the origins of WhereScape, which transitioned from a consulting firm to a software company due to the demand for their pioneering automation tools. Tools like WhereScape significantly enhance developer output, reduce errors, and cut down both costs and operational risks.
Kent added depth to the discussion by sharing insights from his extensive experience in data warehousing and analytics. He highlighted that automation not only simplifies the initial development phases but also significantly eases ongoing maintenance. This shift reduces the time and cost involved in adjustments and upgrades, which are inevitable given the dynamic nature of business requirements.
A key point discussed was the five main beneficial factors of data warehouse automation:
- Cost Reduction: Automation dramatically speeds up data warehousing processes, directly reducing costs.
- Risk Mitigation: Automating repetitive tasks lowers the probability of errors, thus mitigating the risks of errors associated with manual coding.
- Complexity Management: As data sources and technologies proliferate, automation helps manage and simplify complexity. Kent pointed out that the technical complexity of integrating various data sources often explodes without automation, making these integrations manageable and less error-prone.
- Urgency: In fast-changing environments, like those witnessed during the COVID-19 pandemic, the ability to quickly adapt data systems is crucial. Kent emphasized that automation enables businesses to rapidly respond to changing business needs, thus maintaining competitiveness.
- Agility: Automation supports agile practices by enabling rapid prototyping, allowing for immediate feedback and iterative improvements. This agility is essential in modern data environments where requirements can evolve during the development process.
As Kent succinctly puts it, “Automation is not just about making things faster, it’s about making them more reliable and adaptable in the face of changing business needs.” This perspective underscores the transformational impact of automation on data warehousing, highlighting its critical role in contemporary business practices. Together, Patrick and Kent provided a comprehensive overview of how automation transforms data warehousing from a cumbersome and risky business operation into a more manageable, efficient, and responsive practice.
Levels of Automation
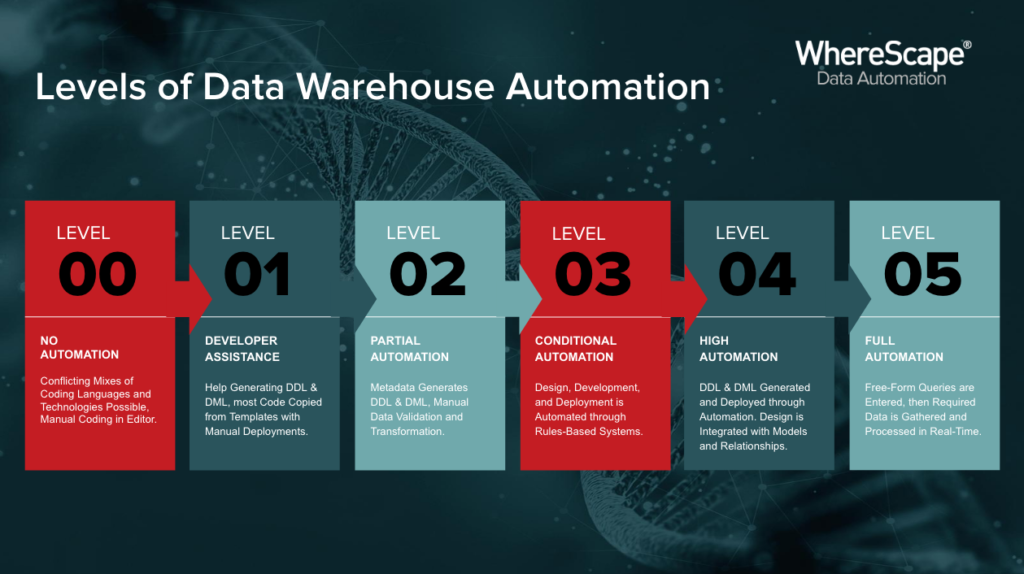
Drawing an interesting parallel with the levels of automation in-vehicle technologies, Patrick introduced a concept after reading about Mercedes-Benz announcing their achievement in reaching Level 3 in automated driving technology, where data warehouse automation could also be segmented into different levels, from basic automation to fully autonomous systems.
This categorization helps in understanding the depth and impact of automation at each stage:
- Level 0: No automation. Tools are entirely manual, similar to driving a car that lacks even basic assists like an automatic transmission.
- Level 1: Basic tools that assist developers without fully automating the process, akin to vehicles with manual controls but some electronic aids.
- Level 2: Some automation where the tools handle simple tasks and workflows but still require significant human interaction for complex decisions and actions.
- Level 3: Conditional automation where tools can handle more complex tasks and decision-making under specific conditions, requiring human intervention in unusual or complex scenarios only.
- Level 4: High automation where the system can perform most tasks and operate independently in most scenarios, but may still require human oversight in highly complex or novel situations.
- Level 5: Full automation, where systems can operate with minimal human input, analogous to fully autonomous vehicles.
Patrick provided insights into how each level affects the workflow and efficiency of data warehousing projects. He discussed current capabilities at WhereScape, placing their solutions between Levels 3 and 4, indicating significant automation yet still requiring some level of human oversight.
Kent added valuable context to this discussion by reflecting on the parallels between automation in cars and data systems. He emphasized that just as higher levels of automation in vehicles reduce the need for driver involvement, higher levels of data warehouse automation reduce the need for constant developer oversight, thereby increasing efficiency and reducing the likelihood of human error. He noted the integral role of comprehensive metadata in supporting high-level automation:
“The automation tool has to have access to this information to know where those source systems are, how to connect to those source systems, what the security protocols are for those source systems, and then go through looking at those data catalogs and say, How how do these things integrate?”
Kent Graziano
Together, they explained the various stages of automation in data warehousing, highlighting the practical applications of each level and underscoring the advantages of progressing towards more automated systems. Their insights reveal a clear trajectory towards higher automation, promising not only increased efficiency but also enhanced reliability and adaptability in the ever-evolving landscape of data management.
Future Perspectives on Data Warehouse Automation
The discussion concluded with a visionary look towards the future, focusing on the potential realization of Level 5 automation in data warehousing. Both speakers speculated that future systems could autonomously integrate data from multiple sources and systems without human intervention. They envisioned a scenario where advancements in AI and machine learning not only automate the integration and management of data but also enhance the analytical capabilities of these systems, enabling them to make intelligent decisions and provide insights with minimal human input.
The Need for Human Oversight in Advanced Automation
However, they also acknowledged the current limitations inherent in these technologies. While AI and machine learning are rapidly advancing, both speakers highlighted the importance of human oversight in ensuring data integrity and relevance. They highlighted that, despite the move towards more autonomous systems, human expertise remains crucial in defining the parameters of automation, setting goals, and interpreting outputs that AI systems generate.
Kent added a technical perspective on this potential future, discussing the role of metadata in facilitating higher levels of automation. He emphasized, “The more the higher level automation you want to go, the more important it is that that metadata already exists somewhere, that there’s valid metadata supporting what your data infrastructure looks like.” He elaborated on how enhanced metadata frameworks could support AI systems in understanding data context, thereby reducing errors and enhancing the reliability of automated decisions.
Graziano further stressed the challenges of achieving Level 5 automation, stating,
“Based on some rules, (AI) can make some guesses, but a human then still has to look at it and go, ‘Yeah, that’s the primary key. And those are the right foreign keys.’ So without that metadata there, there’s no way for an AI really to infer it very well, unless it’s doing all the data profiling again, based on some rules. It’s gotta have some rules. It’s gotta have some metadata for us to really get to that.”
Kent Graziano
He stressed the need for ongoing innovation in AI ethics and governance to ensure that as data systems become more autonomous, they also remain transparent, accountable, and aligned with organizational values and objectives.
While Level 5 automation offers exciting possibilities, it also prompts a cautious approach. The integration of AI and machine learning into data warehousing must be handled with a keen understanding of the technology’s capabilities and its implications for data management practices. As these systems evolve, the synergy between human expertise and machine efficiency will likely define the future landscape of data warehousing, ensuring that automation enhances rather than undermines the strategic value of data.
Navigating the Future of Data Warehouse Automation
We explored the transformative journey of data warehouse automation, from WhereScape’s pioneering beginnings to the potential of full automation. As we integrate more AI and machine learning, these technologies promise to revolutionize data warehousing by making systems not only faster but smarter.
Enhance your data strategies by exploring the capabilities of WhereScape RED, WhereScape 3D, and Data Vault Express. Watch our on-demand webinar to discover how these tools can transform your approach to data management. If you’re ready to make your data work harder for you, book a demo with us here.
Want Better AI Data Management? Data Automation is the Answer
Understanding the AI Landscape Imagine losing 6% of your annual revenue—simply due to poor data quality. A recent survey found that underperforming AI models, built using low-quality or inaccurate data, cost companies an average of $406 million annually. Artificial...
RED 10: The ‘Git Friendly’ Revolution for CI/CD in Data Warehousing
For years, WhereScape RED has been the engine that powers rapidly built and high performance data warehouses. And while RED 10 has quietly empowered organizations since its launch in 2023, our latest 10.4 release is a game changer. We have dubbed this landmark update...
The Assembly Line for Your Data: How Automation Transforms Data Projects
Imagine an old-fashioned assembly line. Workers pass components down the line, each adding their own piece. It’s repetitive, prone to errors, and can grind to a halt if one person falls behind. Now, picture the modern version—robots assembling products with speed,...
The Role of Clean Data in AI Success: Avoiding “Garbage In, Garbage Out”
Co-authored by infoVia and WhereScape Artificial Intelligence (AI) is transforming industries across the globe, enabling organizations to uncover insights, automate processes, and make smarter decisions. However, one universal truth remains: the effectiveness of any...
What is a Cloud Data Warehouse?
As organizations increasingly turn to data-driven decision-making, the demand for cloud data warehouses continues to rise. The cloud data warehouse market is projected to grow significantly, reaching $10.42 billion by 2026 with a compound annual growth rate (CAGR) of...
Simplify Cloud Migrations: Webinar Highlights from Mike Ferguson
Migrating your data warehouse to the cloud might feel like navigating uncharted territory, but it doesn’t have to be. In a recent webinar that we recently hosted, Mike Ferguson, CEO of Intelligent Business Strategies, shared actionable insights drawn from his 40+...
2025 Data Automation Trends: Shaping the Future of Speed, Scalability, and Strategy
As we step into 2025, data automation isn’t just advancing—it’s upending conventions and resetting standards. Leading companies now treat data as a powerful collaborator, fueling key business decisions and strategic foresight. At WhereScape, we’re tuned into the next...
Building Smarter with a Metadata-Driven Approach
Think of building a data management system as constructing a smart city. In this analogy, the data is like the various buildings, roads, and infrastructure that make up the city. Each structure has a specific purpose and function, just as each data point has a...
Your Guide to Online Analytical Processing (OLAP) for Business Intelligence
Streamline your data analysis process with OLAP for better business intelligence. Explore the advantages of Online Analytical Processing (OLAP) now! Do you find it hard to analyze large amounts of data quickly? Online Analytical Processing (OLAP) is designed to answer...
Mastering Data Warehouse Design, Optimization, And Lifecycle
Building a data warehouse can be tough for many businesses. A data warehouse centralizes data from many sources. This article will teach you how to master data warehouse design, optimization, and lifecycle. Start improving your data strategy today. Key Takeaways Use...
Related Content
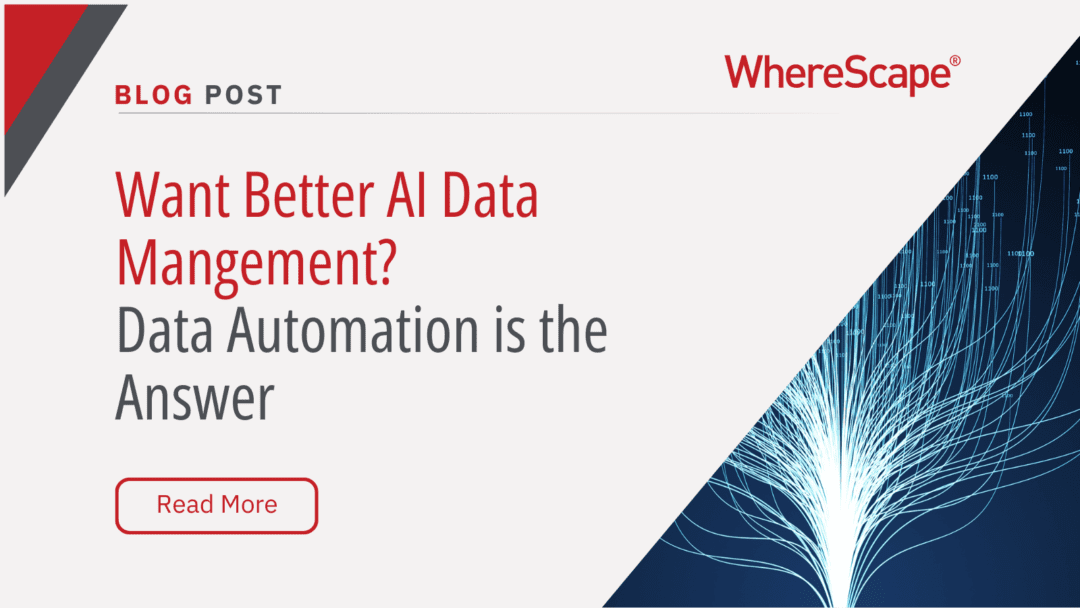
Want Better AI Data Management? Data Automation is the Answer
Understanding the AI Landscape Imagine losing 6% of your annual revenue—simply due to poor data quality. A recent survey found that underperforming AI models, built using low-quality or inaccurate data, cost companies an average of $406 million annually. Artificial...
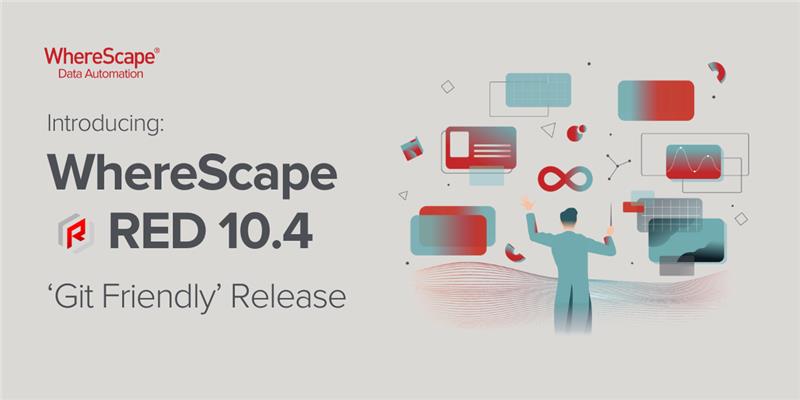
RED 10: The ‘Git Friendly’ Revolution for CI/CD in Data Warehousing
For years, WhereScape RED has been the engine that powers rapidly built and high performance data warehouses. And while RED 10 has quietly empowered organizations since its launch in 2023, our latest 10.4 release is a game changer. We have dubbed this landmark update...
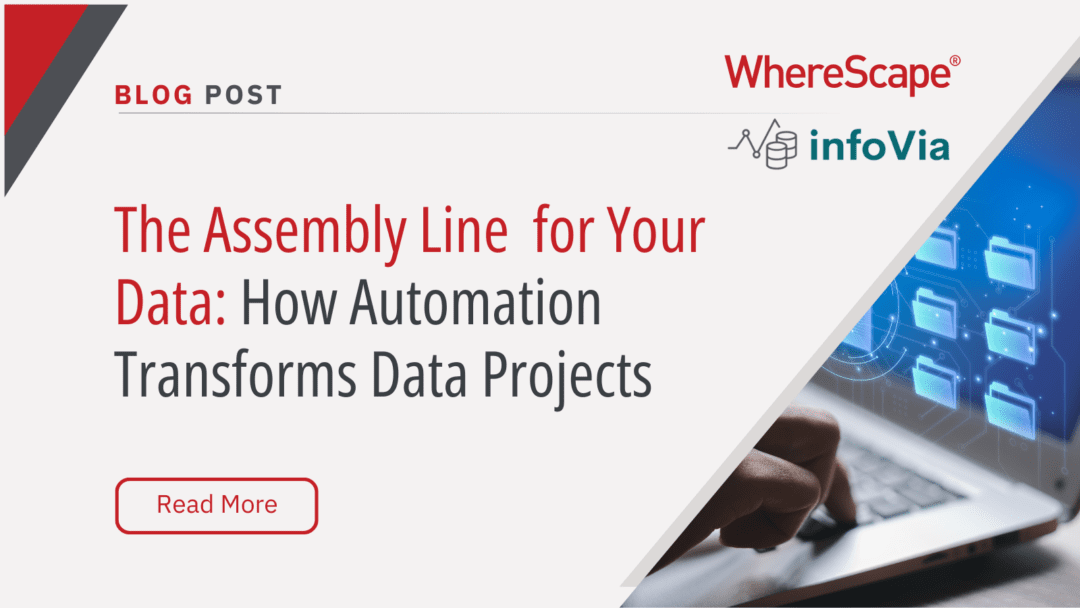
The Assembly Line for Your Data: How Automation Transforms Data Projects
Imagine an old-fashioned assembly line. Workers pass components down the line, each adding their own piece. It’s repetitive, prone to errors, and can grind to a halt if one person falls behind. Now, picture the modern version—robots assembling products with speed,...
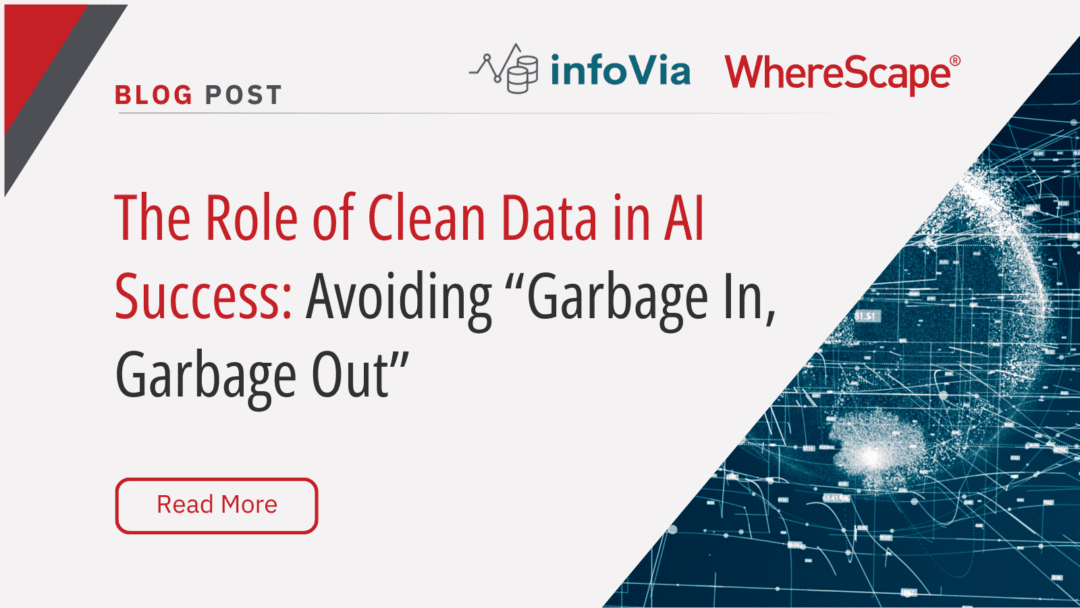
The Role of Clean Data in AI Success: Avoiding “Garbage In, Garbage Out”
Co-authored by infoVia and WhereScape Artificial Intelligence (AI) is transforming industries across the globe, enabling organizations to uncover insights, automate processes, and make smarter decisions. However, one universal truth remains: the effectiveness of any...