Join us for an insightful webinar where we...
Efficient Processing Techniques for JSON and Parquet Semi-Structured Data
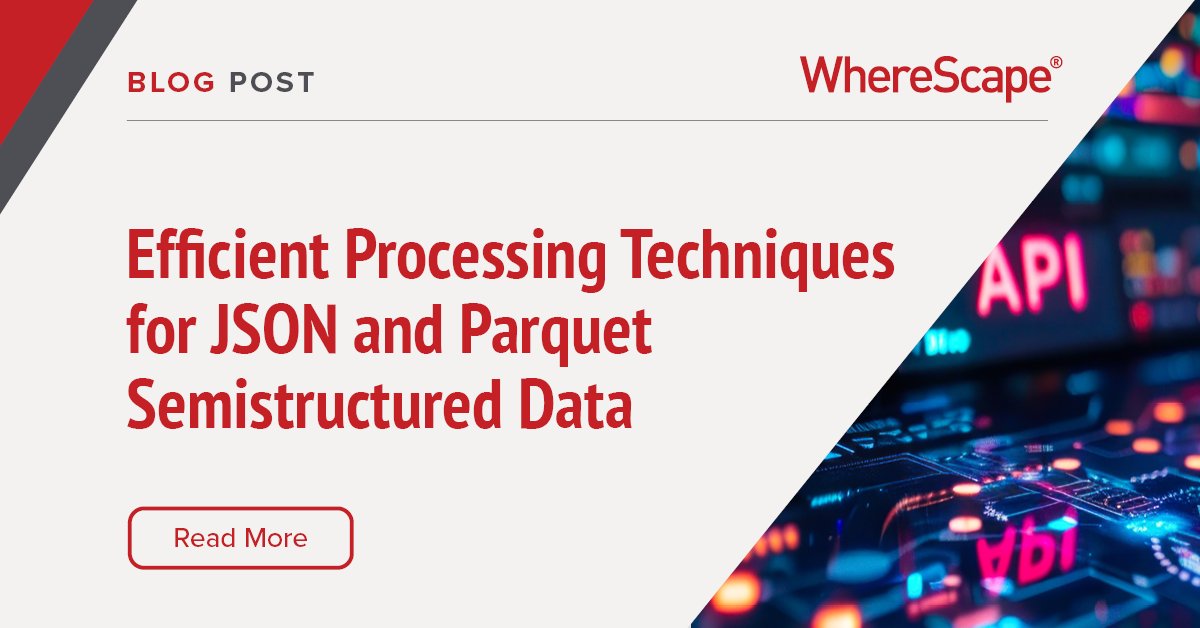
Introduction to Semi-Structured Data and Its Importance
Semi-structured data sits on the spectrum somewhere between traditional database tables and unstructured data. It has organizational properties that make it easier to analyze than raw text, but it doesn’t fit into traditional databases. JSON and Parquet are both examples of semi-structured data.
Semi-structured data stands out for its flexibility and ease of use. Leveraging semi-structured data is essential because it enables businesses to derive actionable insights from complex data sources like logs, IoT devices, and social media interactions, which don’t fit into traditional database structures.
The Role of JSON Data in Today’s Data-Driven World
JSON (JavaScript Object Notation) is a lightweight that is often used because of its flexibility, readability, and widespread support. Because it creates human-readable text to represent complex data hierarchies, it is indispensable in web development and beyond.
A few common applications of JSON data include web APIs and real-time data feeds. JSON facilitates the seamless integration and communication of complex data structures across a diverse array of systems and applications.
Deep Dive: JSON vs. Parquet for Semi-Structured Data
The specific needs of your data application will dictate whether JSON or Parquet is a better fit for your semi-structured data handling. On the one hand, JSON’s strengths lie in its flexibility and ease of use. It is particularly useful for lightweight messaging and web data because of its text-based format.
On the other hand, Parquet is designed with efficiency in mind. Its efficiency of data storage and its high-speed retrieval capabilities make it ideal for large-scale analytics platforms.
Analyzing the Strengths and Weaknesses of JSON
Coming to a deep understanding of the strengths and weaknesses of JSON can help data teams better understand their best options for semi-structured data handling.
Strengths of JSON include:
- Simplicity
- Human readability
- Accessibility in web contexts
- Supports a wide range of data types
- Easy to integrate with many programming languages
On the other hand, weaknesses of JSON include:
- Lack of storage efficiency for large datasets
- Significant overhead due to verbose nature
- Slows down processing
- Increased storage costs
- Not suitable for large-scale analytics platforms
The Advantages of Using Parquet for Data Storage and Analysis
The advantages of Parquet vs JSON mainly focus on applications involving large volumes of semi-structured data. These advantages include:
- A columnar storage format
- Efficient data compression and encoding schemes
- Reduced storage footprint
- Support for advanced optimization techniques such as predicate pushdown
- Query performance
Overall, Parquet is the superior choice when it comes to large-scale analytical workloads.
Transitioning from JSON to Parquet for Data Efficiency
Transitioning from JSON to Parquet can significantly enhance data efficiency, particularly for big data applications. Parquet is a columnar storage file format that offers optimized data compression and encoding schemes. This reduces storage needs and improves read/write speeds, which is especially beneficial for analytics. Overall, Parquet allows for faster querying and data retrieval, which are crucial for efficiently handling large-scale data sets.
Understanding the Conversion from JSON to Parquet
The conversion from JSON to Parquet is a pivotal process for data efficiency. This transition brings a focus to changing formats as well as embracing a more structured, efficient approach to data storage and analysis.
The Technicalities of JSON and Parquet in Data Processing
In order to implement efficient processing practices, data teams must consider the technicalities of JSON and Parquet in Data Processing. JSON is predominantly used in data interchange. In order to manage its hierarchical structure and lack of indexing, it requires careful handling or else performance may be affected.
With Parquet, data teams encounter encoding capabilities that help efficiently process large datasets. Parquet uses a binary file format that also allows for complex nested data structures.
Key Techniques for Managing JSON Data Effectively
In order to effectively manage JSON data, data teams can then take following steps:
- Validate schema
- Use efficient parsing libraries to ensure the speed and integrity of all data
- Implement caching mechanisms
- Leverage stream processing to minimize resource utilization
Optimizing Data with Parquet: Best Practices
For Parquet, the focus shifts to optimizing data through compression and encoding, enhancing read/write efficiency and enabling faster insights from analytical queries. Strategies for data optimization with Parquet include:
- Align data schema with query patterns
- Take advantage of Parquet’s columnar storage format by organizing data such that frequently accessed columns are easily retrievable.
- Implement partitioning of data files based on key attributes that are often used in queries to facilitate faster data retrieval.
- Cluster data within partitions around frequently accessed columns to further enhance query performance.
- Periodically merge smaller Parquet files into larger ones to reduce the overhead associated with managing numerous small files.
- Utilize Parquet’s support for predicate pushdown to perform filtering at the storage level.
- Implement indexing strategies where possible to speed up data retrieval for specific types of queries.
Harnessing the Full Potential of Semi-Structured Data
Organizations can adopt integrated data automation systems like WhereScape in order to fully harness the potential of semi-structured data. WhereScape helps streamline the integration and management of complex data systems, facilitating rapid deployment. With WhereScape’s automation tools, organizations can reduce manual coding time and efficiently extract actionable insights from their data.
With customizable visualization tools, businesses can turn complex data tasks into clear information while maintaining data integrity. This approach enhances decision-making and operational efficiency through automated, intelligent data handling.
Leveraging Webcasts for Advanced Learning: “Efficient Processing Techniques for JSON and Parquet Semi-Structured Data”
Finding educational webcasts can help data teams better understand the nuances of processing techniques and semi-structured data and apply key techniques in their data workflows.
Enhance Your Skills with Our Detailed Webcast
For in-depth demonstrations of the contents of this article as well as practical insights for efficiently processing JSON and Parquet semi-structured data, access our free webcast.
Experience the Power of WhereScape 3D 9.0.3: New Features and Improvements
We’re thrilled to introduce our latest iteration of WhereScape 3D! Version 9.0.3 brings a host of new features and enhancements designed to make your data warehousing journey smoother, faster, and more efficient. Let’s dive into the details of what you can expect from...
Ahead of the Curve: Future Trends in Data Automation and WhereScape’s Pioneering Solutions
The Evolving Landscape of Data Automation As new technologies emerge and existing tools constantly change and improve, the world of data automation transforms rapidly. Even the most well-versed data teams find themselves disoriented and overwhelmed in the face of...
Investing in Data Automation: A Strategic Approach to Business Growth
Unlocking Growth: The Strategic Advantage of Data Automation Organizations reaping the benefits of data automation stay ahead of industry trends and improve the efficiency of their operations and decision-making. Data automation tools offer a strategic advantage for...
Data + AI Summit 2024: Key Takeaways and Innovations
The Data + AI Summit 2024, hosted by Databricks at the bustling Moscone Center in San Francisco, has concluded with remarkable revelations and forward-looking innovations. Drawing over 16,000 attendees in person and virtually connecting over 60,000 participants from...
WhereScape RED 10.1 is Here: Enhanced Scheduling and Customization
We’re proud to announce the highly anticipated WhereScape RED 10.1 is now available, and it’s packed with exciting new features and enhancements designed to make your data warehousing experience more efficient and enjoyable. Let's take a closer look at what’s new and...
Supercharging Data Integration: The WhereScape and Databricks Advantage
The demand for robust data management systems has never been higher, and Databricks has quickly become a favored choice for cloud-based solutions. Its powerful capabilities make it a top contender for managing large-scale data, but when combined with WhereScape's...
Empowering Customer Success: WhereScape’s Comprehensive Support and Training Resources
Enhancing Operational Success with WhereScape’s Support Systems At WhereScape, we understand that a data warehouse is only useful to the extent that it is understood. In order to drive your organization closer to your key goals and objectives, you need full mastery of...
Revolutionizing Day-to-Day Operations: The Power of Automated Data Integration
The Transformational Role of Automation in Data Management Across industries and business stages, organizations of all types manage data in their daily operations. Whether that data entails patient appointments and reminders in a healthcare clinic, student performance...
Gartner® Insights: Microsoft Fabric as a Unified Data & Analytics Platform
Are you ready to revolutionize your data management strategy with a platform that promises to simplify and enhance your operations? According to a Gartner poll, 43% of respondents believe that the data and analytics ecosystem will significantly influence their choice...
WhereScape and YellowFin Attending World of Data in Munich
We are excited to announce that WhereScape and YellowFin will be attending the World of Data conference in Munich on June 6, 2024. This event will bring together data professionals, industry leaders, and technology enthusiasts from around the globe to explore the...
Related Content
Experience the Power of WhereScape 3D 9.0.3: New Features and Improvements
We’re thrilled to introduce our latest iteration of WhereScape 3D! Version 9.0.3 brings a host of new features and enhancements designed to make your data warehousing journey smoother, faster, and more efficient. Let’s dive into the details of what you can expect from...
Ahead of the Curve: Future Trends in Data Automation and WhereScape’s Pioneering Solutions
The Evolving Landscape of Data Automation As new technologies emerge and existing tools constantly change and improve, the world of data automation transforms rapidly. Even the most well-versed data teams find themselves disoriented and overwhelmed in the face of...
Investing in Data Automation: A Strategic Approach to Business Growth
Unlocking Growth: The Strategic Advantage of Data Automation Organizations reaping the benefits of data automation stay ahead of industry trends and improve the efficiency of their operations and decision-making. Data automation tools offer a strategic advantage for...
Data + AI Summit 2024: Key Takeaways and Innovations
The Data + AI Summit 2024, hosted by Databricks at the bustling Moscone Center in San Francisco, has concluded with remarkable revelations and forward-looking innovations. Drawing over 16,000 attendees in person and virtually connecting over 60,000 participants from...