Join us for an insightful webinar where we...
Data Fabric: Streamlining Unified Data Management
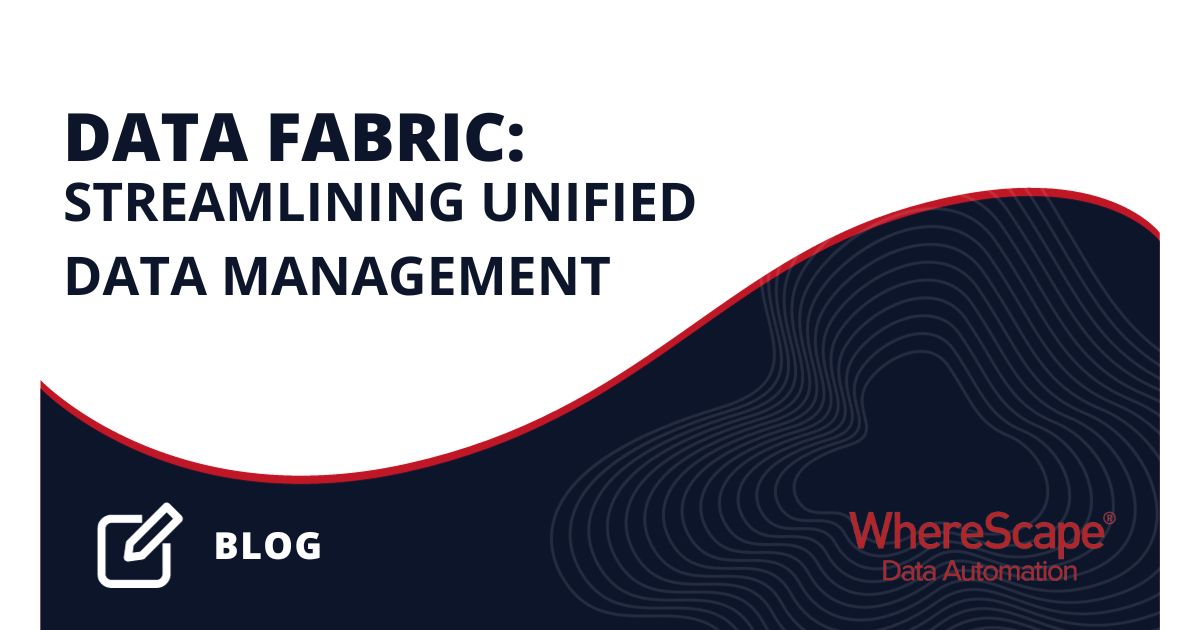
In the dynamic landscape of modern enterprises, the integration of data fabric solutions has emerged as a pivotal strategy to streamline and enhance data processes. These innovative solutions blend diverse data delivery technologies, creating flexible pipelines, services, and semantics under centralized governance. This article delves into the evolution, benefits, challenges, and strategic implementation of data fabrics, shedding light on their transformative role in empowering organizations.
What is a Data Fabric?
The concept of a “Data Fabric” was conceived in the early 2010s, with Forrester introducing the term in 2013. Since then, it has gained widespread adoption among various papers, vendors, and analyst firms. The architects behind this term aimed to design an all-encompassing architecture capable of analyzing data for any type of analysis, ensuring seamless accessibility and shareability.
Data Fabric Benefits
Data fabrics actively assist organizations in addressing complex data challenges by providing frictionless access, fostering data sharing, and facilitating effective data modeling within intricate data environments. By integrating data modeling, a well-constructed data fabric significantly reduces the time taken to access, ingest, integrate, share, and act on data, creating new opportunities for innovation.
Why Choose a Data Fabric?
For enterprises seeking a modern and efficient data management solution, a Data Fabric offers a comprehensive approach to consolidating data from various sources. This not only simplifies data management but also accelerates data processing, enabling faster and more informed decision-making. The scalability of Data Fabric accommodates the ever-increasing volume and variety of data in today’s business landscape, fostering productivity, better decision-making, and a competitive edge.
Implementing a Unified Data Fabric
A fully consolidated analytics architecture through a Data Fabric offers several benefits, including easier data management, enhanced security, reliability, and consistency. By democratizing data and analytical assets, organizations enable easy discovery and navigation of all data assets, leading to a coordinated, documented process of data lineage and usage. This reduction in complexity facilitates the implementation of adaptive, non-technical data bridges across teams.
Businesses need Data Fabric to have access to a secure, efficient, unified environment, and future-proof data solution to make the data accessible to users who need it, with minimal disruption.
Some of the many benefits of a fully consolidated analytics architecture are:
• Easier data management, security, reliability, and consistency: Well-documented metadata simplifies the overall environment.
• Democratization of data and analytical assets: This consists of easy discovery and navigation of all the data assets by all users from a centralized data access mechanism.
• A coordinated, documented process of data lineage and usage: Data redundancy, inaccuracy, senescence, and potential security/privacy breaches can be better controlled here than in a chaotic, undocumented situation.
• Reduction of complexity: The ultimate goal of a Data Fabric is to manage data in a clean and streamlined mindset, enabling organizations to implement adaptive, non-technical data bridges across teams.
How Data Fabric Automates the Data Ecosystem
Data Fabric connects and translates data from various sources, operating in a multi-phase flow of connecting and collecting, accessing and acting, and simplifying and democratizing data. This architecture plays a pivotal role in enhancing machine learning models and building a holistic customer view, providing operational benefits such as simplified data orchestration and automated test data management.
Data Fabric Architecture
The flexibility of Data Fabric architecture works seamlessly with data warehouses, data lakes, and various other data sources, contributing to improving organizational processes. From enhancing machine learning models to building a comprehensive customer view, Data Fabric ensures simplified data orchestration, automated test data management, quick data privacy compliance, comprehensive data administration, and optimized cost of ownership.
Some of the Data Fabric examples include:
- Enhancing machine learning (ML) models: A Data Fabric architecture helps to use ML modes by decreasing the time taken to monitor data pipelines and identify appropriate relations while enhancing the usability of this data across applications and providing controlled access to secure data.
- Building a holistic customer view: A Data Fabric can help organizations gather data from customer activities, therefore adding value to the data such as consolidating real-time data of different sales activities, the time taken to onboard customers, and customer satisfaction KPIs.
Here are some of the operational benefits Data Fabric provides to enterprises:
- Simplified data orchestration: Integrating operators for external databases, business logic, masking, parsing, and streaming.
- Automated test data management: Generating data from production systems to provide high-quality data for testing purposes.
- Quick data privacy compliance: Configuring, managing, and auditing data access requests linked with data privacy regulations.
- Comprehensive data administration: Configuring, monitoring, and administering data using management tools.
- Optimized cost of ownership: Reliance on the in-memory performance of commodity hardware, complete linear scalability, and risk-free integration.
Data Fabric Challenges and Solutions
Enterprises face challenges such as increasing data volumes, multiple locations, complex data formats, and data quality issues. To overcome these challenges, organizations should avoid overloading their architecture with false assumptions, assess metadata maturity, and implement data governance, integration, and consolidation strategies.
Efforts to centralize all data rarely succeed because of a mixture of on-premises and multi-cloud environments which add to the complexity. Moreover, there is an increasing need for regulatory compliance, security, and addressing governance risks, making data discovery more challenging. In such a scenario, implementing data governance, data integration, and consolidation strategies across multiple cloud platforms becomes vital to ensure efficient data management and analysis.
This is where Data Fabric, incorporating master data management, comes to the rescue. Designed to democratize data access across the enterprise at scale, Data Fabric addresses both data silos and the exponential growth in data volumes. By integrating master data management, enterprises can capitalize on these data volumes in a multi-cloud environment while maintaining a secure and compliant data governance strategy.
Data Quality Maintenance
Implementing processes and controls to address data quality challenges is crucial for effective Data Fabric implementations. Data cleansing, transformation, and standardization, coupled with the use of data quality software, ensure high-level data quality maintenance throughout the implementation process.
By automating data quality processes, organizations can identify and correct errors in data, preventing incorrect decisions. As a result, data quality software can play a vital role in helping organizations transform their data quality and gain insights that can help them improve their business operations.
The Relevance of Data Fabric Now
In the face of challenges like limited data access and complex data integration, Data Fabric empowers organizations to leverage their data efficiently. With its scalable and flexible nature, Data Fabric simplifies data governance and management in multi-cloud data landscapes, enabling the creation of a global and agile data environment.
Implementing a Modern Data Fabric Strategy
As data-related challenges compound with growth, a Data Fabric becomes imperative for organizations. This architectural approach ensures data accessibility for relevant users based on their workflows, unleashing the power of data for better decision-making and gaining a competitive edge through hybrid cloud experiences.
Streamline Your Data Analysis with WhereScape for Informed Decision-Making
In conclusion, Data Fabrics represent a transformative force in modern enterprises, offering a holistic approach to data management. By standardizing, connecting, and automating data management practices, organizations can unlock the full potential of their data, gaining deeper insights for informed decision-making.
WhereScape’s Data Fabric solution stands out as a valuable tool, streamlining data analysis and empowering data engineers, data scientists, and business users alike. Click here to request a demo and explore the dynamic capabilities of WhereScape for your organization.
Experience the Power of WhereScape 3D 9.0.3: New Features and Improvements
We’re thrilled to introduce our latest iteration of WhereScape 3D! Version 9.0.3 brings a host of new features and enhancements designed to make your data warehousing journey smoother, faster, and more efficient. Let’s dive into the details of what you can expect from...
Ahead of the Curve: Future Trends in Data Automation and WhereScape’s Pioneering Solutions
The Evolving Landscape of Data Automation As new technologies emerge and existing tools constantly change and improve, the world of data automation transforms rapidly. Even the most well-versed data teams find themselves disoriented and overwhelmed in the face of...
Investing in Data Automation: A Strategic Approach to Business Growth
Unlocking Growth: The Strategic Advantage of Data Automation Organizations reaping the benefits of data automation stay ahead of industry trends and improve the efficiency of their operations and decision-making. Data automation tools offer a strategic advantage for...
Data + AI Summit 2024: Key Takeaways and Innovations
The Data + AI Summit 2024, hosted by Databricks at the bustling Moscone Center in San Francisco, has concluded with remarkable revelations and forward-looking innovations. Drawing over 16,000 attendees in person and virtually connecting over 60,000 participants from...
WhereScape RED 10.1 is Here: Enhanced Scheduling and Customization
We’re proud to announce the highly anticipated WhereScape RED 10.1 is now available, and it’s packed with exciting new features and enhancements designed to make your data warehousing experience more efficient and enjoyable. Let's take a closer look at what’s new and...
Supercharging Data Integration: The WhereScape and Databricks Advantage
The demand for robust data management systems has never been higher, and Databricks has quickly become a favored choice for cloud-based solutions. Its powerful capabilities make it a top contender for managing large-scale data, but when combined with WhereScape's...
Empowering Customer Success: WhereScape’s Comprehensive Support and Training Resources
Enhancing Operational Success with WhereScape’s Support Systems At WhereScape, we understand that a data warehouse is only useful to the extent that it is understood. In order to drive your organization closer to your key goals and objectives, you need full mastery of...
Revolutionizing Day-to-Day Operations: The Power of Automated Data Integration
The Transformational Role of Automation in Data Management Across industries and business stages, organizations of all types manage data in their daily operations. Whether that data entails patient appointments and reminders in a healthcare clinic, student performance...
Gartner® Insights: Microsoft Fabric as a Unified Data & Analytics Platform
Are you ready to revolutionize your data management strategy with a platform that promises to simplify and enhance your operations? According to a Gartner poll, 43% of respondents believe that the data and analytics ecosystem will significantly influence their choice...
WhereScape and YellowFin Attending World of Data in Munich
We are excited to announce that WhereScape and YellowFin will be attending the World of Data conference in Munich on June 6, 2024. This event will bring together data professionals, industry leaders, and technology enthusiasts from around the globe to explore the...
Related Content
Experience the Power of WhereScape 3D 9.0.3: New Features and Improvements
We’re thrilled to introduce our latest iteration of WhereScape 3D! Version 9.0.3 brings a host of new features and enhancements designed to make your data warehousing journey smoother, faster, and more efficient. Let’s dive into the details of what you can expect from...
Ahead of the Curve: Future Trends in Data Automation and WhereScape’s Pioneering Solutions
The Evolving Landscape of Data Automation As new technologies emerge and existing tools constantly change and improve, the world of data automation transforms rapidly. Even the most well-versed data teams find themselves disoriented and overwhelmed in the face of...
Investing in Data Automation: A Strategic Approach to Business Growth
Unlocking Growth: The Strategic Advantage of Data Automation Organizations reaping the benefits of data automation stay ahead of industry trends and improve the efficiency of their operations and decision-making. Data automation tools offer a strategic advantage for...
Data + AI Summit 2024: Key Takeaways and Innovations
The Data + AI Summit 2024, hosted by Databricks at the bustling Moscone Center in San Francisco, has concluded with remarkable revelations and forward-looking innovations. Drawing over 16,000 attendees in person and virtually connecting over 60,000 participants from...