Join us for an insightful webinar where we...
From Data Warehouse Automation to Data Architecture Automation
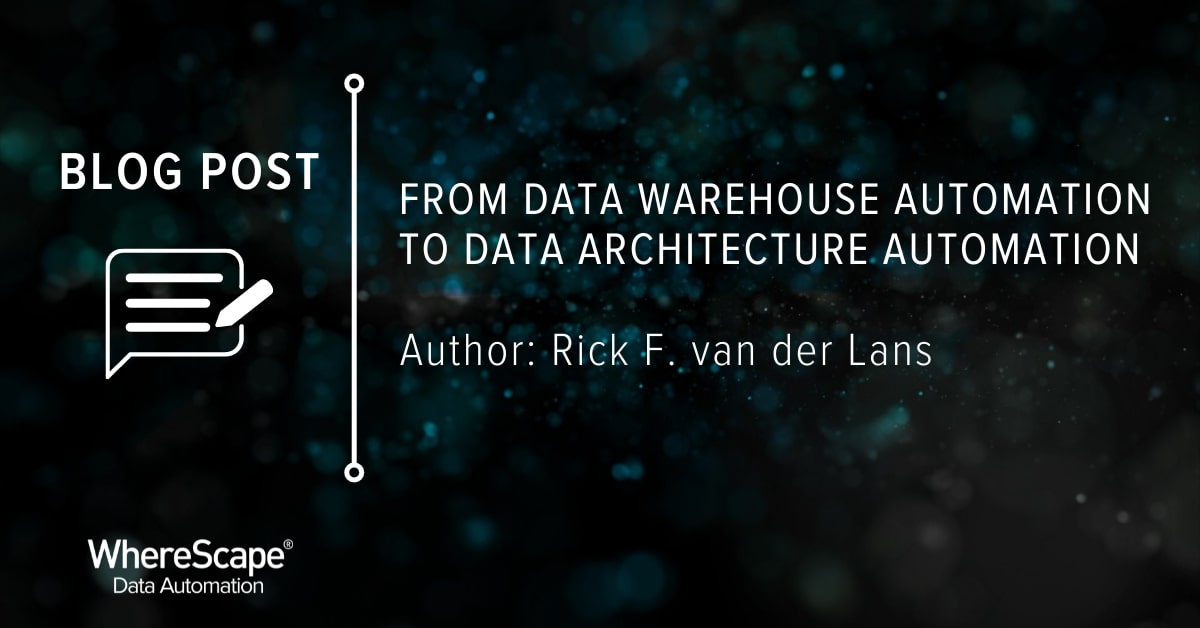
For a long time, the data warehouse architecture was the sole ruler of data delivery to decision-making processes, but not anymore. It now has to share the stage with other data architectures, such as the data lake, data hub, and data lakehouse. Because the data in these new data architectures is structured, organized and used differently, a new breed of generators is required: the data architecture automation tools.
The benefits of using generators are clear. They accelerate development, ease maintenance, create run-time platform independence, improve performance, and so on.
Not every task is suitable for automation and for which suitable generators can be developed. The best tasks suitable for automation are repetitive by nature and can be expressed as formal algorithms that indicate which steps to perform, what to do in special cases and how to react if something goes wrong. In other words, these tasks can be formalized.
Many of the tasks involved in designing, developing and maintaining data warehouse architectures are repetitive and can be formalized, making them highly suited for automation. For example, when an enterprise data warehouse uses a data vault design technique and the physical data marts use star schemas, both can be generated from a central data model including the ETL code to copy the data from the warehouse to the data marts.
Data architecture automation tools can be referred to as the third generation of generators used in automating the development of data architectures to support decision-making processes. The first generation is formed by tools such as ETL, BI and data modeling tools. For example, ETL tools transform high-level specifications to lower-level code to do the actual ETL work, many BI tools can be considered to be generators because they generate SQL statements that extract data from databases, and some data science tools enable data scientists to work at a high conceptual level from which code is generated.
All these generators help to accelerate development and ease maintenance, but they are all limited to generate just one component of an entire data architecture. Therefore, multiple independent generators are required to generate the complete architecture. Since these generators require similar specifications, they are defined multiple times, or in other words, they are duplicated. It is a challenge to keep all these scattered specifications consistent, to ensure that they work together optimally, and to guarantee that if one specification is changed, all the duplicate specifications are changed accordingly.
The principles that apply to generators of individual platform components can be applied to generators of entire data architectures. That is why they were succeeded by the second generation of generators, the data warehouse automation tools that generate entire data warehouse architectures. They do not generate code for one component of the architecture, but for several. Traditional data warehouse automation tools generate, for example, staging areas, enterprise data warehouses, physical data marts, the ETL solutions that copy data from one database to another, and metadata. Several of these tools have been on the market for years and have proven their worth. They all store all the metadata specifications once and reuse them when generating, for example, the data warehouse tables, the data mart tables, and the ETL logic to copy the data.
The main restriction of several data warehouse automation tools is that they only generate traditional data warehouse architectures which can only support a restricted set of data consumption forms.
Today, organizations also want to deploy data hubs, data lakes, and data lakehouses. These are used to support new forms of data consumption. For example, in these new data architectures, data is copied to a data hub and from there to a data warehouse, or the data architecture consist of a data lake that stores data from a data warehouse, transactional databases and external data sources.
Supporting other data architectures requires generators that can be adapted to generate data architectures composed of other types of data stores than those supported by more traditional data warehouse architectures. The term data warehouse automation is probably a misnomer for these tools, it is too restrictive. Data architecture automation tool is more suitable. With the increasing need by organizations to become more data-driven, or in in other words, to use data more widely, effectively and efficiently, the need for generators that can generate any kind of data architecture to support any form of data consumption, the need for adaptable data architecture automation tools has increased accordingly.
Experience the Power of WhereScape 3D 9.0.3: New Features and Improvements
We’re thrilled to introduce our latest iteration of WhereScape 3D! Version 9.0.3 brings a host of new features and enhancements designed to make your data warehousing journey smoother, faster, and more efficient. Let’s dive into the details of what you can expect from...
Ahead of the Curve: Future Trends in Data Automation and WhereScape’s Pioneering Solutions
The Evolving Landscape of Data Automation As new technologies emerge and existing tools constantly change and improve, the world of data automation transforms rapidly. Even the most well-versed data teams find themselves disoriented and overwhelmed in the face of...
Investing in Data Automation: A Strategic Approach to Business Growth
Unlocking Growth: The Strategic Advantage of Data Automation Organizations reaping the benefits of data automation stay ahead of industry trends and improve the efficiency of their operations and decision-making. Data automation tools offer a strategic advantage for...
Data + AI Summit 2024: Key Takeaways and Innovations
The Data + AI Summit 2024, hosted by Databricks at the bustling Moscone Center in San Francisco, has concluded with remarkable revelations and forward-looking innovations. Drawing over 16,000 attendees in person and virtually connecting over 60,000 participants from...
WhereScape RED 10.1 is Here: Enhanced Scheduling and Customization
We’re proud to announce the highly anticipated WhereScape RED 10.1 is now available, and it’s packed with exciting new features and enhancements designed to make your data warehousing experience more efficient and enjoyable. Let's take a closer look at what’s new and...
Supercharging Data Integration: The WhereScape and Databricks Advantage
The demand for robust data management systems has never been higher, and Databricks has quickly become a favored choice for cloud-based solutions. Its powerful capabilities make it a top contender for managing large-scale data, but when combined with WhereScape's...
Empowering Customer Success: WhereScape’s Comprehensive Support and Training Resources
Enhancing Operational Success with WhereScape’s Support Systems At WhereScape, we understand that a data warehouse is only useful to the extent that it is understood. In order to drive your organization closer to your key goals and objectives, you need full mastery of...
Revolutionizing Day-to-Day Operations: The Power of Automated Data Integration
The Transformational Role of Automation in Data Management Across industries and business stages, organizations of all types manage data in their daily operations. Whether that data entails patient appointments and reminders in a healthcare clinic, student performance...
Gartner® Insights: Microsoft Fabric as a Unified Data & Analytics Platform
Are you ready to revolutionize your data management strategy with a platform that promises to simplify and enhance your operations? According to a Gartner poll, 43% of respondents believe that the data and analytics ecosystem will significantly influence their choice...
WhereScape and YellowFin Attending World of Data in Munich
We are excited to announce that WhereScape and YellowFin will be attending the World of Data conference in Munich on June 6, 2024. This event will bring together data professionals, industry leaders, and technology enthusiasts from around the globe to explore the...
Related Content
Experience the Power of WhereScape 3D 9.0.3: New Features and Improvements
We’re thrilled to introduce our latest iteration of WhereScape 3D! Version 9.0.3 brings a host of new features and enhancements designed to make your data warehousing journey smoother, faster, and more efficient. Let’s dive into the details of what you can expect from...
Ahead of the Curve: Future Trends in Data Automation and WhereScape’s Pioneering Solutions
The Evolving Landscape of Data Automation As new technologies emerge and existing tools constantly change and improve, the world of data automation transforms rapidly. Even the most well-versed data teams find themselves disoriented and overwhelmed in the face of...
Investing in Data Automation: A Strategic Approach to Business Growth
Unlocking Growth: The Strategic Advantage of Data Automation Organizations reaping the benefits of data automation stay ahead of industry trends and improve the efficiency of their operations and decision-making. Data automation tools offer a strategic advantage for...
Data + AI Summit 2024: Key Takeaways and Innovations
The Data + AI Summit 2024, hosted by Databricks at the bustling Moscone Center in San Francisco, has concluded with remarkable revelations and forward-looking innovations. Drawing over 16,000 attendees in person and virtually connecting over 60,000 participants from...