Ready to explore Data Vault automation...
Gartner’s Report on Data Hubs, Lakes & Warehouses Highlights Knowledge Gap for Data Managers
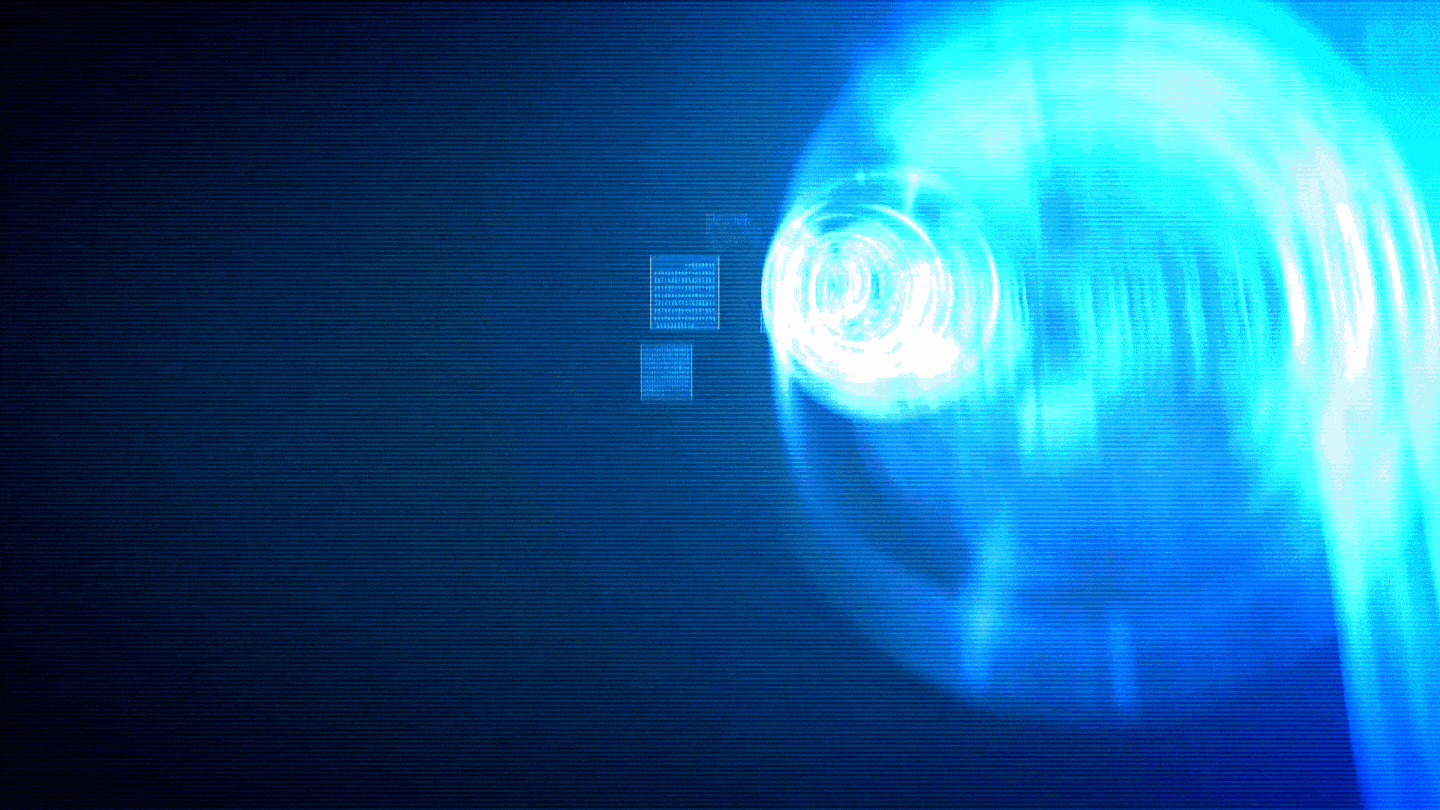
Gartner’s paper, Data Hubs, Data Lakes and Data Warehouses: How They Are Different and Why They Are Better Together, serves as much as a cautionary piece as an informative one. Based on inquiries made to the analyst firm over the past few years, is it apparent that a real gap in knowledge exists when it comes to what these three data structures do and how they should be employed.
“For example, while Gartner client inquiries referring to data hubs increased by 20% from 2018 through 2019, more than 25% of these inquiries were actually about data lake concepts.” (Data Hubs, Data Lakes and Data Warehouses: How They Are Different and Why They Are Better Together by Ted Friedman, Nick Heudecker.)
At the time of the report’s publication in 2020, the percentage of companies using data hubs, lakes and warehouses looked like this:
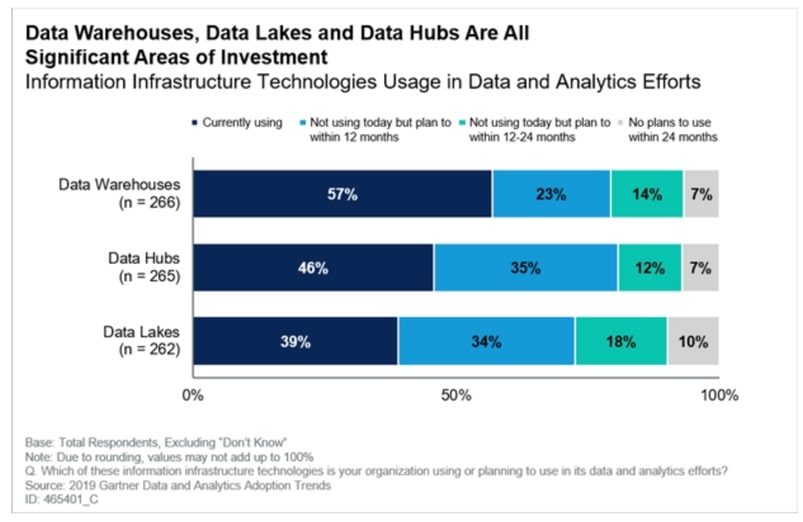
With many companies using all three of these structures already, it’s no exaggeration to say how well companies can understand and harness the potential of data lakes, warehouses and hubs can and will shape their success. This explains the urgency underlying this piece; at present, huge investments are being made by many people who either do not fully understand what each of these three entities does alone and/or how they can be combined most effectively.
How Data Warehouses, Lakes and Hubs Work
Data Warehouses should be used for the analysis of structured data, Data Lakes for analysis of unstructured or semi-structured data, and Data Hubs for communicating the resultant BI to the people who need to act on it. However, many mistakenly think that these three entities do the same thing in different ways, and so are interchangeable. It’s important that business leaders not only understand this for themselves but communicate it throughout the company to democratize the use of data.
Data Lakes and the exploratory technologies that unstructured big data enables are only as useful as your company’s ability to assimilate their findings into a structured environment. This is where the Data Warehouse takes over: a Data Lake can be added as a source to a Data Warehouse, and its data blended with other real-time and batch sources to provide rich, contextualized business insight. Read more on Data Lakehouses here.
Of the three structures, it is ironic that the one managers need to know best is the least understood. The Data Hub is where BI is not only shared but is also available for governance by those responsible for it. As its name suggests a hub also “enables data flow between diverse endpoints”
One of the main recommendations of Gartner’s report is to: “Maximize your ability to support a broader range of diverse use cases by identifying the ways that these structures can be used in combination. For example, data can be delivered to analytic structures (Data Warehouses and Data Lakes) using a Data Hub as a point of mediation and governance.” (Data Hubs, Data Lakes and Data Warehouses: How They Are Different and Why They Are Better Together by Ted Friedman, Nick Heudecker.)
Dealing with Disruption
The report also highlights the need to be agile in how your company can ingest new data from various sources in different formats. Those that can, are able to adapt to disruption and monetize it before their competitors. This supports the use of both a Data Warehouse and lake in conjunction as part of a logical Data Warehouse, and also of an end-to-end automated infrastructure to manage and change it quickly as needed.
While the exponential growth of data makes more insight available, it also means the infrastructure that stores and analyses it becomes necessarily more complex. This infrastructure needs to adapt as new demands emerge (constantly) and as data sources evolve (periodically). It’s a fallacy to think we can create the ultimate data infrastructure that won’t need to be changed.
The Dangers of Ambiguity
Perhaps by reading this piece, data leaders can iron out any ambiguity and potentially make their companies more successful. Misunderstanding also has internal implications in that expectations and reality can be quite different if those leading the data department have different definitions of certain infrastructure than those building and using it day-to-day.
The report is vital reading for data leaders who have even a hint of doubt in their minds of the purpose and role of the Data Warehouse, lake or hub. It could mean the difference between a successful data project, or a failed one in which the roles of the various technologies and staff are not clearly defined.
WhereScape Recap: Highlights From Big Data & AI World London 2025
Big Data & AI World London 2025 brought together thousands of data and AI professionals at ExCeL London—and WhereScape was right in the middle of the action. With automation taking center stage across the industry, it was no surprise that our booth and sessions...
Why WhereScape is the Leading Solution for Healthcare Data Automation
Optimizing Healthcare Data Management with Automation Healthcare organizations manage vast amounts of medical data across EHR systems, billing platforms, clinical research, and operational analytics. However, healthcare data integration remains a challenge due to...
WhereScape Q&A: Your Top Questions Answered on Data Vault and Databricks
During our latest WhereScape webinar, attendees had fantastic questions about Data Vault 2.0, Databricks, and metadata automation. We’ve compiled the best questions and answers to help you understand how WhereScape streamlines data modeling, automation, and...
What is Data Fabric? A Smarter Way for Data Management
As of 2023, the global data fabric market was valued at $2.29 billion and is projected to grow to $12.91 billion by 2032, reflecting the critical role and rapid adoption of data fabric solutions in modern data management. The integration of data fabric solutions...
Want Better AI Data Management? Data Automation is the Answer
Understanding the AI Landscape Imagine losing 6% of your annual revenue—simply due to poor data quality. A recent survey found that underperforming AI models, built using low-quality or inaccurate data, cost companies an average of $406 million annually. Artificial...
RED 10: The ‘Git Friendly’ Revolution for CI/CD in Data Warehousing
For years, WhereScape RED has been the engine that powers rapidly built and high performance data warehouses. And while RED 10 has quietly empowered organizations since its launch in 2023, our latest 10.4 release is a game changer. We have dubbed this landmark update...
The Assembly Line for Your Data: How Automation Transforms Data Projects
Imagine an old-fashioned assembly line. Workers pass components down the line, each adding their own piece. It’s repetitive, prone to errors, and can grind to a halt if one person falls behind. Now, picture the modern version—robots assembling products with speed,...
The Role of Clean Data in AI Success: Avoiding “Garbage In, Garbage Out”
Co-authored by infoVia and WhereScape Artificial Intelligence (AI) is transforming industries across the globe, enabling organizations to uncover insights, automate processes, and make smarter decisions. However, one universal truth remains: the effectiveness of any...
What is a Cloud Data Warehouse?
As organizations increasingly turn to data-driven decision-making, the demand for cloud data warehouses continues to rise. The cloud data warehouse market is projected to grow significantly, reaching $10.42 billion by 2026 with a compound annual growth rate (CAGR) of...
Simplify Cloud Migrations: Webinar Highlights from Mike Ferguson
Migrating your data warehouse to the cloud might feel like navigating uncharted territory, but it doesn’t have to be. In a recent webinar that we recently hosted, Mike Ferguson, CEO of Intelligent Business Strategies, shared actionable insights drawn from his 40+...
Related Content
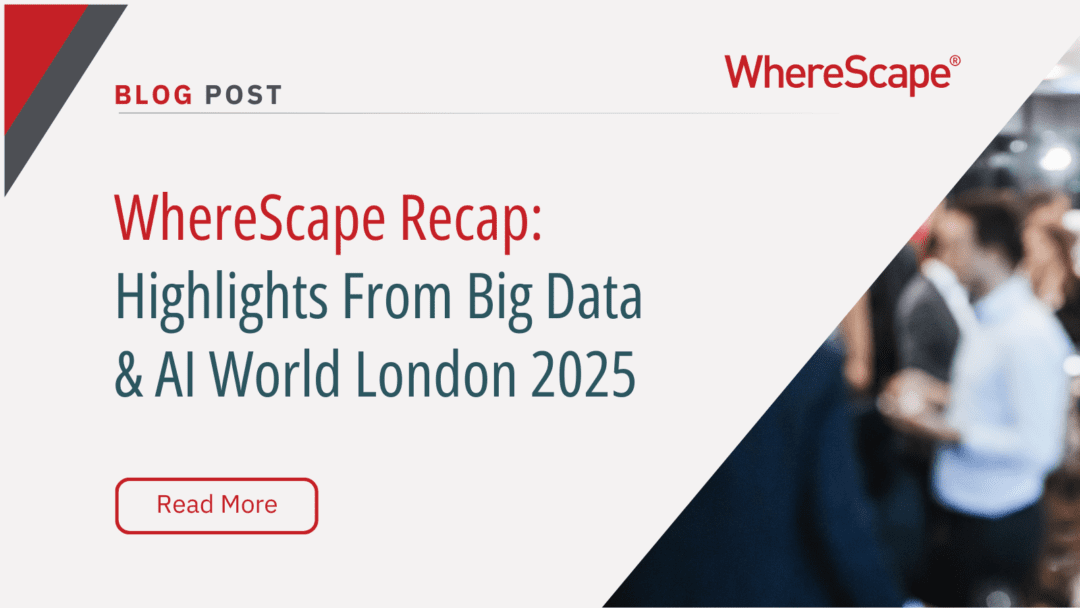
WhereScape Recap: Highlights From Big Data & AI World London 2025
Big Data & AI World London 2025 brought together thousands of data and AI professionals at ExCeL London—and WhereScape was right in the middle of the action. With automation taking center stage across the industry, it was no surprise that our booth and sessions...
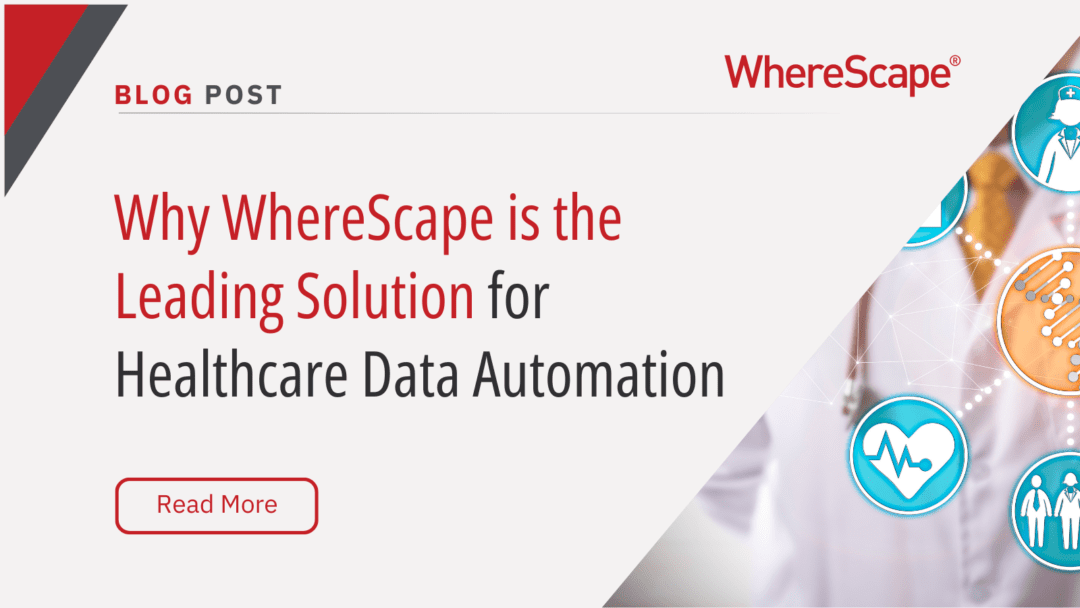
Why WhereScape is the Leading Solution for Healthcare Data Automation
Optimizing Healthcare Data Management with Automation Healthcare organizations manage vast amounts of medical data across EHR systems, billing platforms, clinical research, and operational analytics. However, healthcare data integration remains a challenge due to...
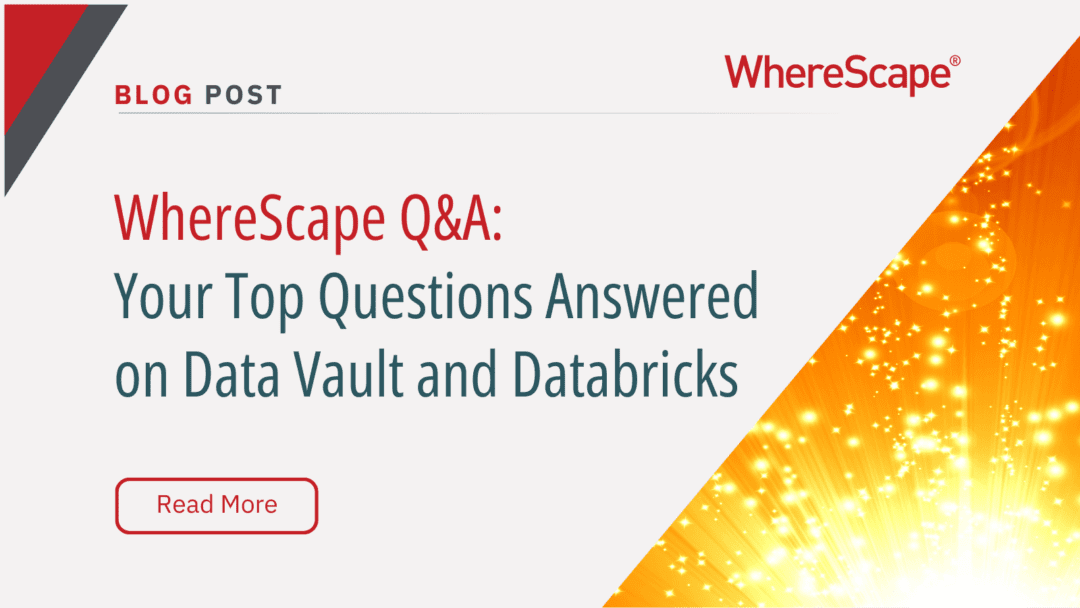
WhereScape Q&A: Your Top Questions Answered on Data Vault and Databricks
During our latest WhereScape webinar, attendees had fantastic questions about Data Vault 2.0, Databricks, and metadata automation. We’ve compiled the best questions and answers to help you understand how WhereScape streamlines data modeling, automation, and...
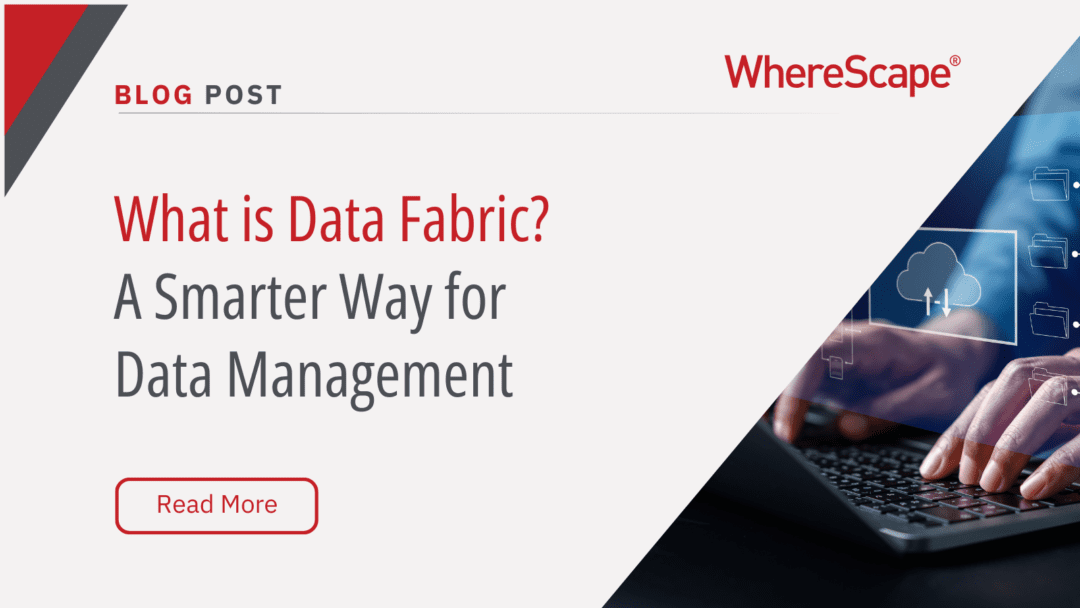
What is Data Fabric? A Smarter Way for Data Management
As of 2023, the global data fabric market was valued at $2.29 billion and is projected to grow to $12.91 billion by 2032, reflecting the critical role and rapid adoption of data fabric solutions in modern data management. The integration of data fabric solutions...